Designing Ethical Technology Requires Systems for Anticipation and Resilience
AI doesn’t just impact speed and costs — it can affect trust, organizational reputation, and the strength of relationships with key stakeholders.
News
- Customer Centricity Summit & Awards Explores Brand-Customer Relationships in KSA
- Data & Analytics Summit in Saudi Arabia will Explore Critical Issues in AI Era
- GITEX Global 2024 to Showcase Global Innovation, Investment, and Cybersecurity Trends
- The Perfectly Imperfect Start of Disruptive Innovations
- GovTech Conclave to Explore Cutting-Edge Solutions for Modern Governance
- New Report Shows Cautious Optimism Among Enterprises Adopting AI
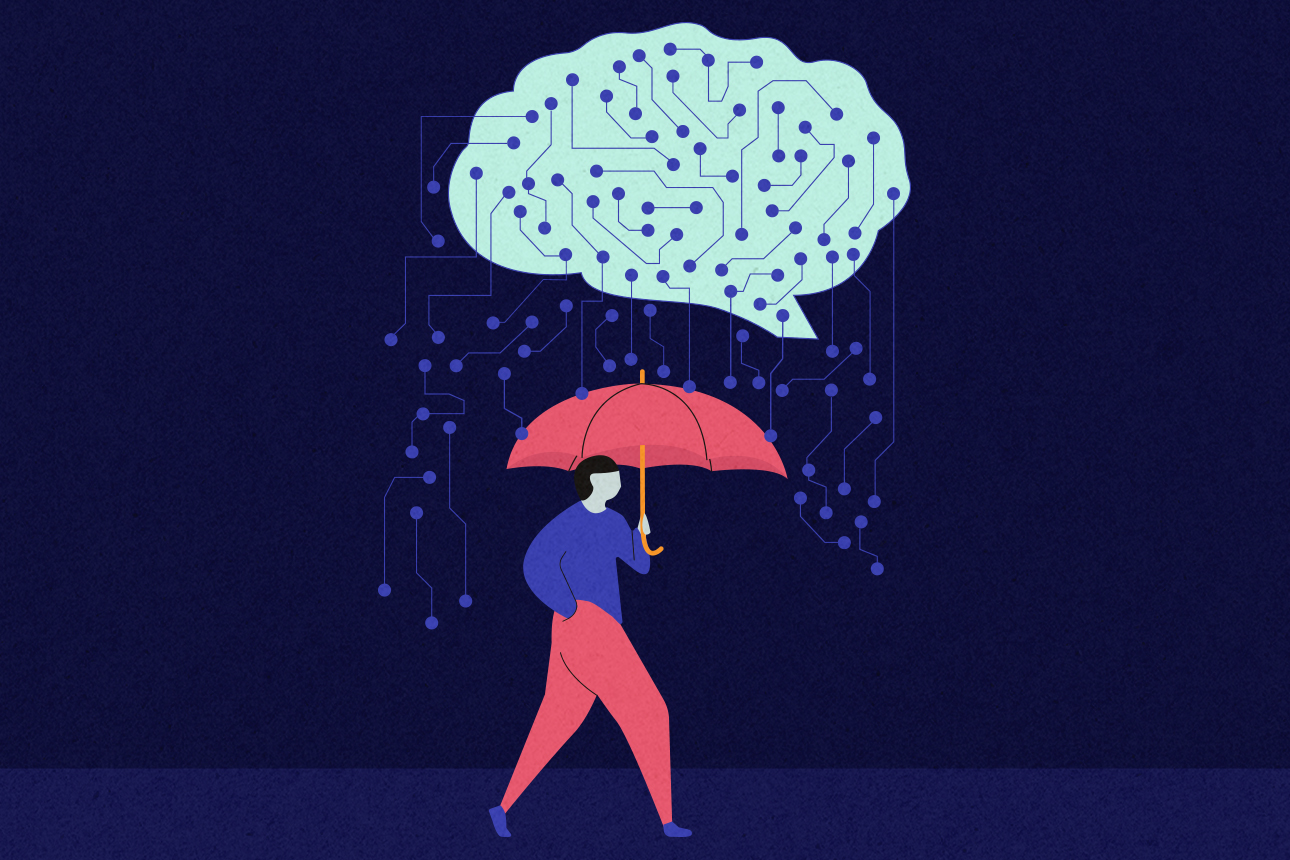
Image courtesy of Laura Wentzel
Concerns about the responsible use of technology are growing as use cases and applications become more sophisticated and ubiquitous across organizations and society. Within the context of AI, recruiting technology company HireVue began experimenting with facial recognition algorithms to filter job applicants based on their facial movements and tone of voice in 2019. This year, the company dropped the technology amid concerns of bias and discrimination against applicants, but organizations will continue to pursue emerging technologies that can help them address persistent managerial questions about hiring, performance, and resource allocation. The scale and speed at which such technologies offer answers to those questions thrust organizational leaders into new territory that has the potential for both higher rewards and higher risks.
More efficient and cheaper computer processing and storage, distributed networks, and cloud-based computing allow for faster, more complex calculations, reducing the amount of time and human intervention needed for a company to evaluate a worker, a credit score, or even a medical diagnosis. In addition to speed, AI impacts the scale of outcomes by standardizing the decisions and actions of multiple individuals across an organization, potentially scaling both consistency and bias. AI also affects second- or third-order stakeholders. For example, the use of cheating-detection software affects not only the company’s direct customers (the school system purchasing the service) but also students and their families who are subject to the AI system’s decisions.
Together, the increased speed and scale of emerging technologies can make ethical lapses more likely, more costly, and harder to recover from. To reduce ethical lapses, organizations need two kinds of systems: systems for anticipation and systems for resilience. In this article, we will examine frameworks for introducing these systems in organizations. We will draw on AI and data-driven examples from the real world, but these principles can be applied to a variety of emerging technologies.
Creating Systems for Anticipation
Anticipating unintended consequences and outcomes requires that leaders take the time to learn about and imagine likely sources of error before the technology is deployed. When ethical lapses are prevented or remedied in the design phase, organizations can avoid reputational and financial damages. Effective anticipation requires managers to do the following:
Define success in broader terms. AI doesn’t just impact speed and costs — it can affect trust, organizational reputation, and the strength of relationships with key stakeholders. Leaders should simultaneously define the goals they want AI to achieve (such as consistency) and the relevant guardrails and values (such as transparency and fairness) that teams need to stay within while developing or using the AI solution.
AI doesn’t just impact speed and costs — it can affect trust, organizational reputation, and the strength of relationships with key stakeholders.
Look out for common lapses. Companies developing AI tools and techniques should keep a running log of mistakes that AI has made so that they can work to reduce that source of error in their own development. Conferences in computer science offer an opportunity to understand the known issues with AI across industries and to exchange strategies for anticipating ethical lapses. We know enough about disparate impact and fairness in the application of AI to routinely test for and prevent these lapses.1
Another common potential lapse is AI’s impact on the distribution of power and privilege in society. AI can maintain unjust social practices, such as longer criminal sentences for minorities, or it can mitigate those unequal outcomes. Developers need to understand how their solutions affect power and privilege in society — and they can do so only if they take the time to inquire about and discuss potential impacts. Conversations about potential lapses should occur early in the development process and also be repeated at key points to minimize unforeseen risks getting through the cracks.
Create constructive conflict in development. Another proven anticipatory strategy is to have internal teams look for errors so that they can write better code. These protocols go by different names, such as red team/blue team and adversarial development, and work just as well for detecting ethical lapses as they do for finding errors in code. Without explicit strategies to foster constructive conflict, important information about risks can go unspoken and overlooked by the development team, particularly when trust and psychological safety are low among team members.
Use risk as a stage gate. The pharmaceutical industry uses a series of escalating clinical trials to prove safety and minimize risk before a drug can be sold. A potential therapy has to pass specific gates at specific stages to gain further investment. Similarly, AI tools can be tested in sandboxes, with data sets that are not live, to find and fix errors before moving on to subsequent stages of development. For example, one source of failure for AI is the difference between the training set and the actual data that it is analyzing. One stage gate might be to conduct an analysis measuring the discrepancy between the training set and “real” data, to build confidence in the AI system’s suggestions.
Creating Systems for Resilience
While companies can work toward anticipating mistakes that are foreseeable, they should also aim to address errors that cannot be foreseen due to the speed and scale of different technology projects. In these cases, companies need systems for resilience, or a set of practices that can help them quickly bounce back from errors.
Release solutions as perpetual betas. First, don’t assume that mistakes won’t occur — they will. Be willing to revise, update, and improve technological solutions. Think of your AI as being in a perpetual beta version that will be improved over time, and have a plan in place to identify, judge, and fix mistakes. This can be an appeals process or a “bug bounty” program for both internal and external stakeholders.
Prepare for transparency. Stakeholders have their own reasons for wanting to understand algorithms — so be ready to justify your choices when things go wrong. When Amazon designed a program to judge and fire delivery drivers, contracted drivers were not provided with an explanation or what they considered to be a viable appeals process. Transparency may be needed for stakeholders to understand the outcomes and to contest or appeal the AI decision.2 Finally, transparency may be needed to know which humans are responsible for how the AI works. For Amazon’s managers at the time, according to a Bloomberg article, “it was cheaper to trust the algorithms than pay people to investigate mistaken firings so long as the drivers could be replaced easily.”
Account for repairs. When mistakes occur, the organization will incur costs to protect data and repair damaged stakeholder relationships. It’s imperative that leaders take the time to prepare for these scenarios and not assume those costs away.
Identify errors in implementation. Employees and researchers need to feel safe in order to identify and report errors. Consider how you can increase psychological safety to protect whistleblowers and have organizational leaders normalize speaking up about ethical issues and concerns. The identification of errors by outsiders is a gift to improve your program: Bug bounty programs reward those who take the time to identify an organization’s errors. Disabling researchers’ accounts and issuing cease-and-desist orders do not engender trust.
Be aware of social embeddedness. All mistakes are not equal, and some have more controversial historical and social contexts. When a medical prioritization algorithm downgraded the priority of Black patients with severe illness, the program was rightly criticized for having a disparate impact and worsening the situation for those already facing discrimination. When choosing how to deploy AI, be aware of how the AI program distributes power and how it could further disenfranchise individuals.
The use of new technology by organizations involves both promise and perils. To realize more of the promise and minimize the perils, organizational leaders need to ensure the presence and robustness of systems for anticipation and resilience. The more we can catch mistakes early on that we are not currently sensing and anticipating, the better we can prepare to bounce back from mistakes that we cannot foresee.
One way to think about developing and implementing AI is that it is similar to developing policy at scale. A policy is a set of rules that affects millions of people in ways we might not be able to foresee. Just like policy, AI makes value judgments about who it will affect and how — and those judgments can have unintended and unforeseen consequences. By understanding the value judgments made during the design process and the moral implications of AI as it is deployed, organizations can deliver on the promises of new technology while fostering trust with stakeholders.
References
- S. Barocas and A.D. Selbst, “Big Data’s Disparate Impact,” California Law Review 104, no. 3 (June 2016): 671-732.
- D.N. Kluttz, N. Kohli, and D.K. Mulligan, “Shaping Our Tools: Contestability as a Means to Promote Responsible Algorithmic Decision-Making in the Professions,” in “After the Digital Tornado: Networks, Algorithms, Humanity,” ed. K. Werbach (Cambridge, England: Cambridge University Press, 2020), 137-152.