Does GenAI Impose a Creativity Tax?
LLMs can boost worker productivity, but outputs may reflect less human creativity and originality.
News
- Customer Centricity Summit & Awards Explores Brand-Customer Relationships in KSA
- GITEX Global 2024 to Showcase Global Innovation, Investment, and Cybersecurity Trends
- The Perfectly Imperfect Start of Disruptive Innovations
- GovTech Conclave to Explore Cutting-Edge Solutions for Modern Governance
- New Report Shows Cautious Optimism Among Enterprises Adopting AI
- Majority of CISOs Feel Their Organizations are Unprepared for Cybersecurity Regulations
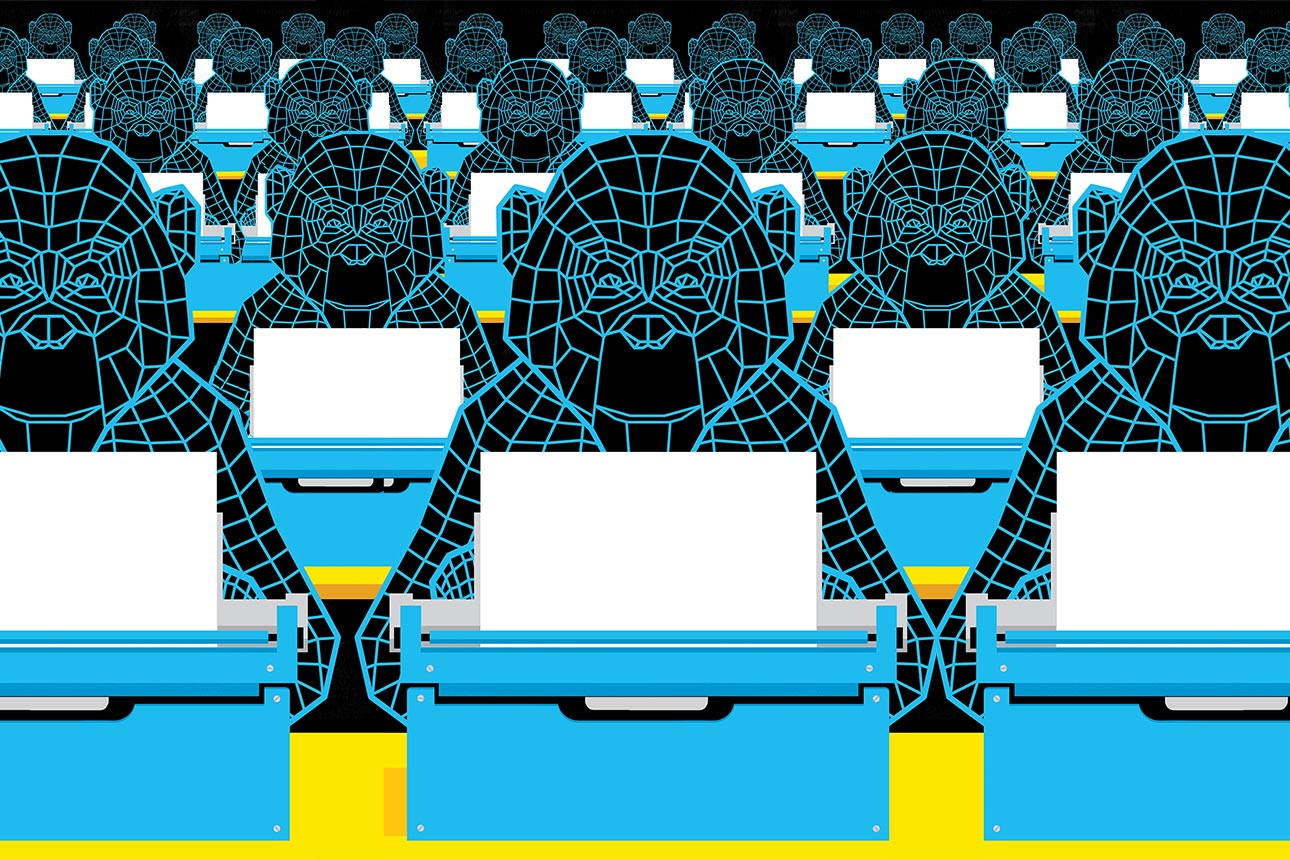
Chris Gash/theispot.com
Generative AI systems that model language have shown remarkable proficiency at a variety of tasks, and employees have embraced them to speed up writing and software development work in particular. The productivity boosts promised by these tools, such as ChatGPT, are leading many managers to incorporate them into workflows. However, our research reveals that the potential efficiency improvements come with a potential downside.
Overreliance on AI may discourage employees from expressing their specific know-how and coming up with their own ideas, and could also result in increasingly homogenized outputs that limit the advantages of employee diversity. In the long term, this could diminish innovation and originality. Managers seeking to gain efficiencies via large language models (LLMs) will need to help employees thoughtfully balance productivity and creativity in their collaboration with AI.
The Trade-Off Between Originality and Effort
AI-generated content impressively mimics the linguistic fluency of human-created content but typically lacks a specific user’s stylistic choices and the original thinking that they would naturally express when accomplishing the task without AI. Aligning AI outputs to successfully capture the intention of human outputs can require iterative and time-consuming prompt refinement that users may decide is not worth it if the AI’s early output is considered good enough. Thus, users face a decision: Invest time in customizing generative AI suggestions to progressively reflect more of their unique style and know-how — a process that can eat up productive time — or settle for somewhat suboptimal first drafts.
Consider a team of software engineers collaborating on a large-scale software project. As they work on the code base, each team member will make coding and documentation decisions that are in line with agreed-upon standards but are also driven by each individual’s own experience and preferences regarding object architecture, function naming, testing choices, and so on. Just as writers of prose aim to craft brilliant turns of phrase, software engineers strive to develop elegant and original solutions to coding problems.
When productivity is prioritized, LLM-based tools such as GitHub Copilot make it easy to quickly generate a draft or autocomplete large blocks of code. This can save a lot of time, given that the tools often write decent code and can quickly improve existing code. However, the AI’s first draft might not reflect the team’s best practices or an engineer’s know-how and style. While engineers can refine their AI prompts or edit the code manually to improve it so it’s more faithful to their intent, doing so will slow them down. However, neglecting to do so can have negative implications for future productivity: Later, when a programmer needs to come back to the code to fix a bug or make improvements, the costs and effort of addressing any shortcomings may be significantly higher. Indeed, research has found that individuals often struggle to review and adapt code generated by AI; in some cases, it might be more efficient to start from scratch if changes are needed.
The consequent risk for managers is that putting too much focus on productivity goals and hard-to-meet deadlines may encourage employees to eschew the extra effort and simply accept more generic outputs. This could have significant negative repercussions: A 2023 study found that LLM-based coding tools can diminish code quality and maintainability.
This scenario is one already faced by workers leaning on generative AI tools to do their writing for them. AI integration with enterprise software suites has made it easy to task LLMs with writing emails, generating reports, or designing presentation slides. In such cases, users face a similar trade-off between accepting output that they might judge to be suboptimal in terms of accuracy or writing originality, and making the extra effort to coax better results out of the tools via prompt refinements. Time pressure is likely to figure large in these user choices. There is no free lunch here: The more time users spend editing the content themselves or refining iterative prompts, the closer the tool’s output will be to users’ preferences and standards. If they routinely accept the initial AI output, the organization will accumulate content — or code — that doesn’t really reflect the know-how and expertise for which employers value talented performers.
In a working paper, we introduced a simple mathematical model tailored to re-create and capture key aspects of human-AI interactions. Below, we describe what it teaches us about the potential consequences of broad AI adoption.
Putting Creativity at Risk
Our research suggests that as people try to balance the trade-off between getting optimal output and working most efficiently when interacting with AI, diversity of thought and creativity tend to be lost. Defaulting to the tool’s unmodified output can result in content that is more homogenous than what would be created by individual humans. If everyone’s emails were written by Microsoft Copilot, for example, they would likely all sound similar. Such homogeneity at scale can put at risk the originality and diversity of ideas and content that are essential for growth and innovation.
This issue of homogenization intensifies when AI-generated content is used to train subsequent AI models. The rational use of this new technology and the AI’s learning process can create a feedback loop, potentially leading to a homogenization death spiral — in which the AI-generated content loses diversity. This concern is heightened as more AI-generated content finds its way into the pools of data used to train LLMs, whether it be proprietary organizational content or material on the internet. If the web becomes saturated with AI-generated content and we increasingly incorporate AI into our workflow and content generation processes, the creativity and diversity of our ideas will be significantly reduced. Some researchers have made the case that LLMs training on more LLM-generated content than human-generated content could even lead to the collapse of LLM models.
But at this point, because AI can competently generate a great deal of routine content, it may seem that losing diversity of thought is of little consequence, especially given the potentially large efficiency gains. However, the habit of defaulting to LLM outputs could have far-reaching implications for innovation, which depends on originality and creativity. Managers must balance the focus on productivity gains with ensuring that AI tools enhance rather than limit the ideas and perspectives expressed in work products.
There are several ways that managers can gain generative AI’s productivity benefits while also preserving creativity and diversity of thought. First, they should rethink productivity expectations. When evaluating the potential use of generative AI for a given task, managers should consider the nature and requirements of the task and how much oversight or original thought employees are expected to contribute. In some cases, employees may need more time to complete the task with AI.
Enhancing human-AI interactions by enabling users to more easily guide, amend, and correct model output can play a crucial role in their success. For example, retrieval-augmented generation uses external knowledge bases to improve output accuracy. Comprehensive training in prompt engineering should also make it easier for users to convey their own ideas to shape more-original LLM outputs.
Historically, shifts in business, such as automation and offshoring, have transferred the burden of labor and routine tasks to machines or external parties. In turn, this has enabled businesses to increase their productivity and lower their costs. In contrast, while generative AI technology also promises productivity enhancements and reduced costs, it affects businesses in a different realm: that of ideas, content, and innovation. It can lessen our cognitive load in tasks like drafting routine documents or analyzing long reports. However, as we’ve argued above, there are risks to outsourcing too much of our own original or critical thinking. We promote the use of AI as an assistant that enriches our lives and work rather than a substitute that erodes the richness of our individuality and the diversity of our thoughts.
To mitigate these concerns, it is essential for leadership to guide their teams in using AI tools thoughtfully. Managers should encourage their employees to authentically express their distinct perspectives and actively contribute their creativity to the company. This will not only ensure that AI systems are better utilized for realizing efficiency gains while maintaining originality; it will also guard against the potential pitfalls of a homogenous, AI-influenced culture. Cultivating a balanced relationship between humans and AI, where both parties mutually complement each other, will be pivotal in navigating the evolving landscape of AI-driven production and creation within our businesses.