Effective Data Management is Key to Unlocking the Middle East’s AI Potential
As MENA enterprises look to profit from advances in artificial intelligence, demand for trusted, secure data has intensified. How can organizations prioritize data integrity and transparency to unlock AI's full potential?
News
- Gulf Nations Fast-Track AI Ambitions, UAE Leads Regional Readiness, says BCG Report
- AI Professionals form a Redefined Workforce. But Systemic Roadblocks Persist, Survey Finds
- AI-Driven Scams Surge as Microsoft Blocks $4 Billion in Fraud Attempts
- Identity-based Attacks Account for 60% of Leading Cyber Threats, Report Finds
- CERN and Pure Storage Partner to Power Data Innovation in High-Energy Physics
- CyberArk Launches New Machine Identity Security Platform to Protect Cloud Workloads
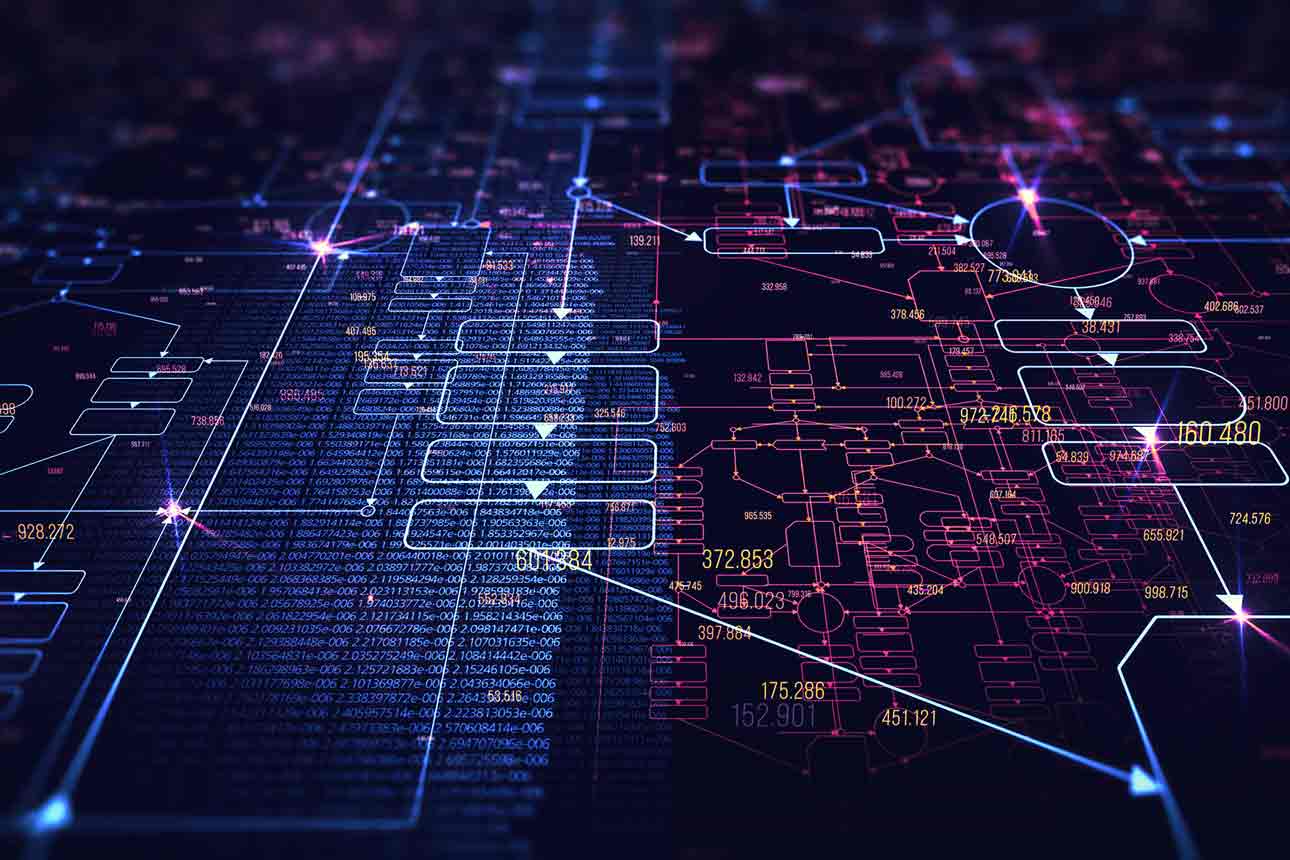
[Image source: Krishna Prasad/MITSMR Middle East]
More enterprises in the Middle East are chasing the golden goose, artificial intelligence (AI), with PWC forecasting a $320 billion dividend for the region by 2030. However, failure to consider the technology’s underlying infrastructure could immobilize hasty AI adopters.
Data is at the core of that gilded infrastructure. Everything begins with data, whether it’s automating document processing, analyzing customer behavior to optimize inventory, or parsing satellite imagery to track environmental changes. AI can transform businesses only when their data is efficiently mined and analyzed.
Wendy Batchelder, Salesforce’s Chief Data Officer, frames it succinctly: “The AI revolution is actually a data revolution, and a company’s AI strategy is only as strong as its data strategy, with trust at its core.”
Regional business leaders agree. According to Salesforce’s recent State of Data and Analytics survey of more than 10,000 analytics, IT, and business leaders across 18 countries, the vast majority (94%) of UAE analytics and IT leaders recognize that advances in AI make next-generation data management a high priority.
“Data is the fundamental building block for any AI solution,” agrees Steve Fernandes, Senior Manager, Solutions Engineering at Confluent. “AI applications, in general, depend heavily on data as the fuel that powers their algorithms and enables them to learn, adapt, and make predictions or decisions.”
A Flood of Dirty Data Degrades AI’s Performance
While enterprises have accumulated a surfeit of data from sensors, apps, and customer interactions, it often needs to be more accurate, consistent, and relevant. This dirty data makes developing and deploying AI models more difficult, degrades their performance, and can lead to incorrect classifications or errors.
“Dirty data is a major problem, as it undermines the reliability of AI models and leads to flawed decision-making,” explains Ramprakash Ramamoorthy, Director of AI Research at ManageEngine, an enterprise IT service management company with operations across the region.
Insiders say that uneven digital capabilities within an organization can compound problems arising from untrustworthy data—a common state of affairs in MENA enterprises.
“Organizations in the region are facing digital imbalance across departments. For example, some departments, such as IT or marketing, are very digitally mature, while a department, such as legal or facilities management, is at the lower end of the digital maturity spectrum. AI does not like data in silos, and better digital maturity can lead to less dirty data and higher quality AI,” Ramamoorthy says.
He shares the case of a UAE-based insurer’s struggles with incorrect and outdated data following April’s record rains. The company had invested in a customer-facing digital claims platform and an AI-powered approval system. However, because its legal department was slower to adapt, flood claim policy changes weren’t quickly updated. The AI relied on outdated policy language, leading to inaccurate assessments and potential claim miscalculations.
Discrepancies between the digital interface and the outdated data powering the AI tool confused and frustrated the company’s customers and employees alike. Potential knock-on effects could surface in the form of financial losses from inaccurate claim assessments and reputational damage.
Damage control measures required the insurer to issue communications clarifying its flood claims policy for customers and employees, update legal policies digitally and integrate them into the AI system, and, over the longer term, improve cross-departmental communications while investing in digital literacy training across the organization.
“The inconsistency eroded trust in the AI system itself, with the root cause being stale data,” he says. “Even advanced AI systems can be undermined by outdated processes in a single department, impacting the customer experience and the effectiveness of the technology.”
Without quality data, AI algorithms lack the material they need to learn, adapt, and generate usable business insights.
Then there are issues around privacy, says Haider Aziz, Managing Director of Middle East, Turkey, and Africa at AI platform data company VAST Data. The New York-headquartered company partnered with UAE cloud services provider Core42, formerly G42 Cloud, to build a central data foundation for a global network of AI supercomputers last August.
“The MENA region faces a complex landscape where balancing data-driven innovation with privacy protection remains a critical challenge,” he says.
While there is growing recognition of the importance of data quality, particularly for social inclusion and citizenship rights, data protection legislation continues to evolve. While some countries have established frameworks, others are still in the early stages. This can create challenges for safeguarding personal data, he explains. In particular, national security agencies and private companies must balance data access with user privacy.
“Finding the right balance is crucial for building trust and fostering responsible data use in the region,” Aziz says.
Next-gen Data Management Strategies to Support AI Use Cases
As the region presses forward with AI, local entities must focus on advanced data management practices to ensure that the data used to train and feed AI systems is accurate, reliable, and transparently sourced.
Ramamoorthy outlines a few next-gen data management measures for regional entities if they are to unleash the full benefits of AI technologies, ensuring that the insights and decisions derived from it are trustworthy and valuable.
- Data governance: Establish clear policies and ownership of data assets to ensure consistency and accountability.
- Data profiling and cleansing: Regularly assess data for errors and inconsistencies and proactively address them.
- Data lineage tracking: This method tracks the origins and transformations of data for better understanding and control. It is especially valuable in the MENA region, where data often traverses multiple systems and languages.
- Bias detection and mitigation: Use tools and techniques to identify potential biases in datasets and implement corrective measures. This must be a continuous process to avoid concept drift.
- DataOps: Implement agile methodologies to streamline data management, such as those in software development.
Beyond those recommendations is the human element.
Fernandes suggests fostering a collaborative culture of trust and learning. “Especially if we look at the regional aspect, cross-functional collaboration among the teams, including data scientists, engineers, and legal professionals, creates effective data management practices, leveraging local expertise and addressing cultural sensitivities, thereby building trust in AI-driven solutions.”
Clean and trustworthy data is crucial to maximizing the benefits of the investments, ensuring reliable outcomes, enhancing efficiency, and driving innovation. Without robust data management strategies, AI algorithms risk producing inaccurate insights and flawed decisions – and that golden, billion-dollar dividend could remain little more than a fairy tale.