How GenAI is Redefining Public Sector Efficiency in the Middle East
Key industry leaders discuss strategies, challenges, and use cases for integrating genAI in the public sector by private players.
Topics
News
- Customer Centricity Summit & Awards Explores Brand-Customer Relationships in KSA
- GITEX Global 2024 to Showcase Global Innovation, Investment, and Cybersecurity Trends
- The Perfectly Imperfect Start of Disruptive Innovations
- GovTech Conclave to Explore Cutting-Edge Solutions for Modern Governance
- New Report Shows Cautious Optimism Among Enterprises Adopting AI
- Majority of CISOs Feel Their Organizations are Unprepared for Cybersecurity Regulations
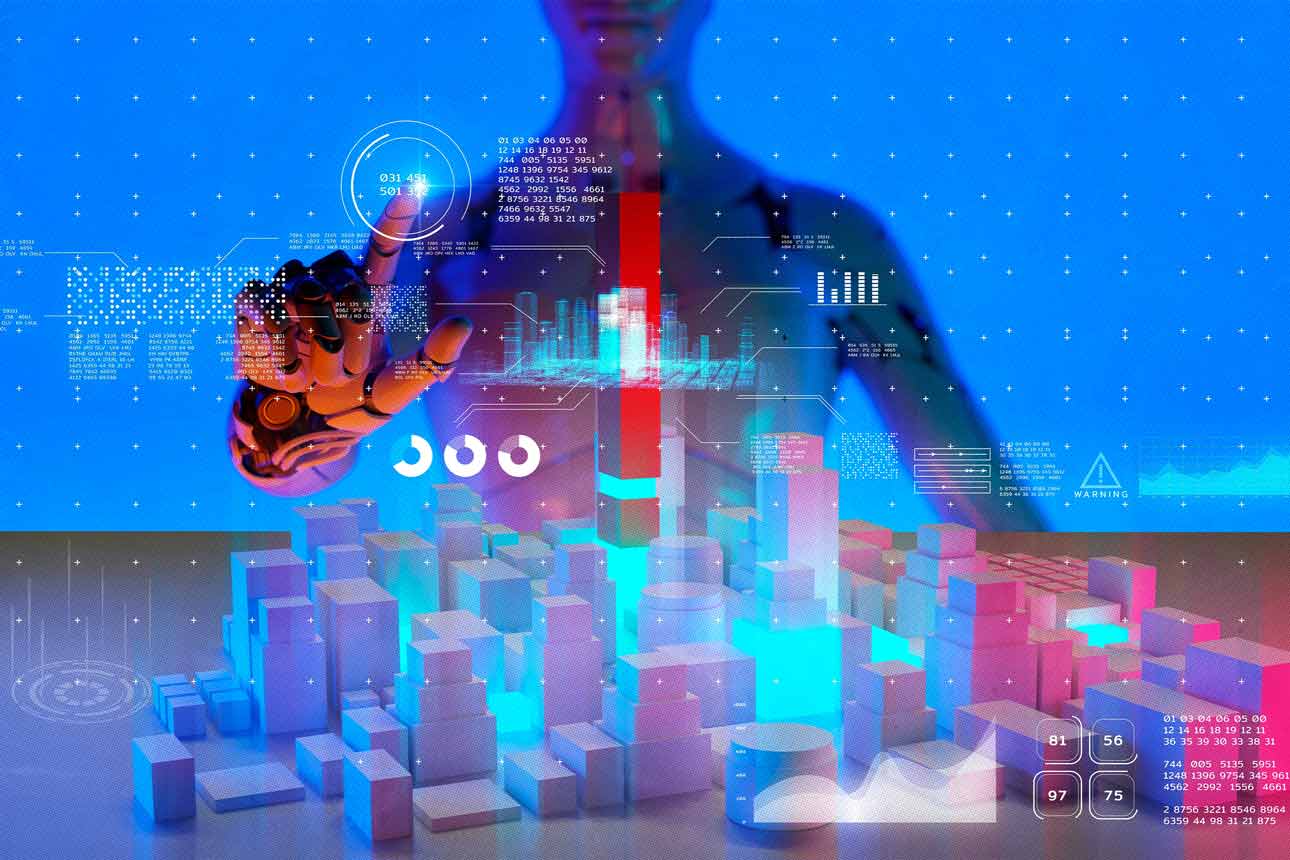
[Image source: Krishna Prasad/MITSMR Middle East]
Abu Dhabi (10), Dubai (12), Riyadh (25), Doha (48), Mecca (52), Jeddah (55), Medina (74), Muscat (88), Al Khobar (99). The numbers represent the cities’ rankings in this year’s IMD Smart City Index, which evaluated 142 cities globally based on economic and technological factors and the “human dimensions” of smart city development.
A key driver behind these rankings is the region’s commitment to integrating emerging technologies like the Internet of Things (IoT), generative AI (GenAI), and digital twins into city infrastructure to enhance efficiency, improve services, and inform data-driven decision-making. According to a report by GlobalData, the smart cities market generated a revenue of $262.5 billion in 2023 and is projected to grow at a compound annual growth rate (CAGR) of 17.2% through 2027.
Building a smart economy – governance, people, mobility, and environment – requires a multi-layered and phased approach. Achieving this vision also requires enhanced collaboration and trust among various stakeholders.
The challenge, however, lies in integrating this transformative, rapidly evolving technology into complex government infrastructures.
The stakes are high, with public trust, data security, and operational continuity hanging in the balance. For private companies, the key to successful AI integration in government systems is an approach combining strategic planning, robust security frameworks, real-world testing, and continuous human oversight.
A Layered Approach to AI Integration
Government systems are often complex and sensitive, making AI integration a delicate process. Cloudera’s Manasi Vartak advocates for a layered approach, emphasizing that AI should augment rather than replace human decision-making. She highlights the use of AI in tasks such as rapid document search and email assistance, with the caveat that human oversight is essential to correct AI-generated outputs, ensuring safety while enabling continuous improvement.
“Extensive real-world testing should precede deployment, followed by continuous monitoring and evaluation to promptly address any unexpected issues ,” the chief AI architect adds.
Rami Mourtada, Partner & Director of Digital Transformation at Boston Consulting Group (BCG), echoes the need for a “strategic and methodical approach.”
The first step for government agencies, he advises, is to clearly define their objectives and comprehend the necessity of leveraging Large Language Models (LLM). Acknowledging that LLM technology, despite its potential, is prone to errors, such as producing unfounded or incorrect information, is crucial in objectively assessing the risk-benefit ratio. Once the objective is set, identify potential AI applications and focus on a few high-impact use cases. “By developing pilot projects for real-world testing, organizations can foster a culture of experimentation and learning,” Mourtada adds.
As AI becomes a cornerstone of digital government transformation, Jaimen Hoopes, Vice President of Product Management at Forcepoint, calls for adopting “robust frameworks and unified security solutions” to successfully and safely integrate GenAI into existing systems. “Defining clear policies for data handling, access controls, and ensuring compliance with regulatory requirements is critical to preventing unauthorized data sharing,” says Hoopes.
Advanced security technologies such as Data Loss Prevention (DLP) and Data Security Posture Management (DSPM) should be implemented to monitor and protect sensitive government data. These solutions ensure data integrity, prevent leaks, and secure AI platforms from cyber threats. As compliance requirements evolve, governments must adopt a unified security approach to ensure GenAI adoption aligns with Zero Trust principles.
Islam Rashad, Head of Cyber Security Solutions Engineering at World Wide Technology (WWT), adds another dimension – a comprehensive system assessment before AI deployment. “Government agencies must first identify areas where AI can enhance efficiency and then develop a customized integration plan that ensures seamless interoperability, incorporating robust security measures to protect sensitive data.”
Top Challenges for Private Firms Deploying AI in Government Settings
“Deploying AI solutions in government settings presents private companies with a range of nuanced challenges that must be meticulously navigated to ensure successful implementation,” says Mourtada.
The digital transformation expert, who has worked with dozens of prominent private and public sector clients across industries in the US, Europe, and the Middle East, highlights the complexity of security and compliance, coupled with the integration difficulties associated with legacy systems, the lack of high-quality data and AI literacy within organizations.
Hoopes concurs that the bureaucratic processes and employees’ resistance to change hinder the adoption of new technologies while also stressing the critical need for user training. Compounding this at a practical level, says Rashad, is the approval and financial constraints.
1. Compliance Complexity: The difficulty of ensuring the security and compliance of the technology within legacy systems and rigid compliance structures stands out as a significant barrier. Governments operate under strict legal frameworks designed to protect privacy and data security, which can introduce complex hurdles for AI deployment. These legal and regulatory obligations necessitate a careful approach to how AI technologies are developed, deployed, and managed, underscoring the critical importance of compliance from the outset.
2. Integration Issues: Another hurdle is integrating new AI technology with existing IT systems within government operations. The public sector often relies on diverse systems and technologies, some of which may be outdated or incompatible with the latest AI solutions. Achieving seamless integration requires a deep understanding of these legacy systems and a strategic approach to ensuring that new AI technologies can function effectively within this established infrastructure. This could also require migration to more modern infrastructures, where diligent compliance criteria and value-based planning should be implemented to evaluate the optimal migration paths with the least disruption.
3. Data Quality: Compounding these integration and compliance challenges is the lack of access to high-quality data for model training. Indeed, limited data accessibility and low quality are among the key challenges in data and AI enablement. Given that approximately 45% of Smart City applications require cross-industry consolidated data, and organizations lose, on average, $15 million annually due to poor data management, the significance of this challenge cannot be overstated. For AI solutions to deliver meaningful outcomes, they necessitate high-quality, clean, and well-structured data. However, the vast and varied nature of data managed by governments, often set back by inconsistency, inaccuracy, or a lack of standardization, poses a formidable challenge to achieving the data quality required for effective AI implementation.
4. AI Literacy and Change Resistance: Insufficient AI literacy throughout the organization, alongside resistance, opposition, and fear about AI’s impact on jobs, adds another layer of complexity. Cultivating an understanding of AI’s potential, mitigating concerns related to job displacement, and fostering a culture that embraces technological advancement become empirical in ensuring that AI solutions can be effectively adopted and utilized within government settings.
Adding to Mourtada’s take on resistance within public institutions, Hoopes says, “Bureaucratic processes and fear of disruption may hinder the adoption of new technologies.” Teams must prioritize employee education by ensuring folks understand what can and can’t be shared with GenAI, and stay apprised of the malware and phishing campaigns that GenAI has the potential to power up.
5. Funding and approval constraints: For WWT, the biggest challenges included obtaining GPU export approvals on a client-by-client basis, ensuring access to high-quality datasets for training AI models, integrating AI with outdated legacy systems, navigating strict regulatory environments, overcoming staff resistance to change, and securing sufficient funding for AI projects within budgetary constraints.
6. User Training: User awareness and training are key to enhancing data security. By educating users about the potential risks associated with GenAI and encouraging best practices, enterprises, and government agencies can be empowered to play a responsible role in ensuring data security and ensuring that AI tools are used safely and effectively.
Strategic Factors for AI Adoption
To navigate the unique complexities of government operations when developing AI strategies, Mourtada highlights three pivotal factors:
1. Impact & Productivity Gain: The potential productivity gains from deploying Gen AI in the public sector are substantial. BCG projects these gains could be valued at up to $1.75 trillion per year by 2033. For instance, Saudi Arabia could see an annual productivity benefit of $56 billion, and the UAE might gain $11 billion over the next decade. This immense value proposition underscores the importance of integrating Gen AI tools within government functions, which could result in both short and long-term wins. Specifically, governments could see diffused productivity improvements ranging from 10% to 20%, alongside notable efficiency gains of 30% to 50%, showcasing the transformative power of Gen AI in enhancing public sector operations.
2. Infrastructure Readiness: This encompasses a significant IT transformation requiring tooling, processes, and talent development investments. Achieving organizational and technical maturity is indispensable, including but not limited to establishing a centralized codebase, and comprehensive documentation of configuration management databases and knowledge bases, alongside streamlined software and DevOps toolchains. A pragmatic approach, starting with a “walk first” strategy, involves the incubation of Gen AI within priority agencies. This allows for cultivating internal “ambassadors” to foster awareness, empower data governance, and champion responsible AI practices across functions.
Real-world Application Example
Felicien Mathieu, Chief Technologist EMEA, Telecom & System Integrators at WWT, says the company deployed a wide range of AI use cases across government delivery programs in the hardware domain, platform domain, and AI use case.
It has helped clients architect the High-Performance Computing Modernization Program (HPCMP). In the defense sector, for example, WWT integrated cloud-based high-performance computing (HPC) solutions into existing infrastructure to enhance computing power for Supercomputing Resource Centers, all while controlling hardware costs.
3. Responsible AI and Ethics: This aspect should spearhead the development and deployment policies of GenAI systems. The policies should clearly articulate an unwavering commitment to ethical principles, particularly in sensitive areas like healthcare, where these systems must prioritize patient privacy and safety. Furthermore, establishing stringent governance mechanisms for GenAI by classifying AI applications and instituting detailed certifications for high-risk AI systems will pave the way for ethically sound development practices.
Crisis Management Examples
In the AI application space, Mathieu says the company implemented Machine Learning (ML) to tackle complex security challenges across the government. WWT collaborated with government entities to apply AI to specific use cases such as border security, cybersecurity, and domestic security. These initiatives were designed to enhance operational efficiency and solve pressing security concerns.
During the COVID-19 pandemic, it integrated and deployed multiple Nvidia DGX SuperPOD systems to accelerate urgent research. Furthermore, WWT engineered and integrated one of the world’s most advanced Nvidia supercomputers at the University of Florida, positioning it as a leading research hub.
A fourth factor that other experts highlight is the scalability and continued stakeholder engagement in projects.
4. Scalability and Stakeholder Engagement: With the expansion of smart and megacities, new developments are inevitable, requiring government technology solutions to scale their functionality, user base, and data processing capabilities without significant changes to the underlying architecture. This scalability is essential for governments to adapt to evolving citizen needs and manage resources efficiently.
For instance, e-government platforms that facilitate citizen engagement and service access must be capable of handling increased traffic and expanding service offerings. IoT solutions for traffic management, waste management, and energy use can grow alongside cities, enhancing efficiency and sustainability. Public safety applications supporting emergency response and disaster management should also be designed to scale during crises, ensuring governments remain prepared and responsive.
“The scalability requirements for government agencies are often similar to those of the largest enterprises,” says Vartak, a computer science PhD from MIT and the founder of a Machine Learning Operations (MLOps) platform that enables data scientists and ML engineers to manage and operate AI-ML models at scale. For example, a solution that works for a Top-5 Global bank is likely scalable enough for most government use cases.
“We design scalable solutions to meet the evolving needs of government operations, engage all relevant stakeholders in the planning and implementation process, and focus on projects that deliver tangible benefits to the public, enhancing government services,’’ adds Rashad.
Mathieu, who has led multi-million cloud transformation projects, also shares an example of how scalable solutions can address large-scale challenges. The technology services provider supported the Abu Dhabi Department of Economic Development, where it implemented a food security real-time platform that integrates cloud deployment, MLOps platforms, and business use cases to provide a single view of key metrics and KPIs for the region.
He also shares an example from the oil and gas industry. AI-powered analytics have helped identify trends and patterns in oil & gas data, leading to more informed growth decisions. The AI-driven automation of routine tasks has also improved service delivery by reducing processing times and increasing accuracy, allowing public sector employees to focus on more strategic activities.
However, government use cases tend to be more diverse than commercial ones, says Vartak. At Cloudera, the focus is on building customizable individual components that can be assembled into different solutions. The modular approach allows the company to tailor AI technologies to meet various government agencies; specific and varied needs, ensuring both scalability and adaptability.
Talking about modular AI solutions — separate, interchangeable components or modules — that can be independently developed, updated, or replaced without affecting the entire system, Mathieu says the company develops reference architectures with partners like Nvidia and Dell. These solutions range from AI node setups for initial adoption to large-scale training infrastructure, delivering fully integrated AI systems, known as SuperPODs, which provide exaflops of computational power.
WWT has also started deploying internal ChatGPT-like systems using Retrieval Augmented Generation (RAG) architectures. These systems integrate with curated datasets, enabling more accurate, data-driven insights while minimizing AI hallucinations, and improving decision-making and operational efficiency.
10-20-70 Framework
To successfully deploy AI at scale and convert it into business impact, BCG recommends organizations consider a 10-20-70 framework, focusing 10% on algorithms, 20% on technology and data, and 70% on people and processes.
- Algorithms: The focus should be on crafting new algorithms and advancing the scientific foundation underpinning them. Given the anticipated growth in AI models’ size and capabilities within the next three to five years, selecting the optimal balance between performance needs and cost becomes increasingly important.
- Technology and Data: Ensure the deployment of suitable tech stacks and the imperative to direct the correct data into the appropriate systems. This necessity often leads to simplifying legacy systems and adopting new AI platforms designed to meet evolving AI requirements. Additionally, the increasing significance of data necessitates the development of capabilities to manage unstructured information efficiently.
- People and Processes: The emergence of new roles, processes, and operating models necessitates a robust change management framework. This includes attracting and upskilling of talent and augmenting existing skill sets to align with the new demands brought about by AI integration.
Addressing Critical Infrastructure and Regulatory Concerns
GenAI systems deployed in government settings must be designed with a deep understanding of the public infrastructure. “Applications deployed in public sector settings should minimize the risk of severe consequences, such as financial losses or human harm,” Vartak says.
Moreover, maintaining the accuracy and reliability of AI models over time is crucial, particularly when dealing with dynamic data, such as intelligence or sensor data. Mechanisms must be in place to ensure AI models are regularly updated to reflect the latest information, thereby ensuring decision-makers can rely on accurate outputs.
But considering the pace at which these technologies are evolving, how do public sector clients determine which AI systems and LLMs to invest in and what options to consider?
This is where the concept of an “AI Testing Ground Lab” becomes invaluable, says Rashad. For instance, WWT’s AI Proving Ground provides a safe and secure sandbox, allowing clients/partners to experiment, test, and innovate with cutting-edge AI hardware and software. The controlled environment de-risks decision-making, ensuring the right AI infrastructure and LLM models are chosen, while the testing and validation phases ensure that AI platforms perform reliably within a governmental context.
Government agencies’ stringent security and compliance requirements often necessitate running software on-premises rather than in the cloud, which can be difficult for companies accustomed to cloud-based solutions. Cloudera addresses this challenge by offering software that operates seamlessly in hybrid environments—both on-premises and in the cloud—and has passed rigorous security and compliance controls, ensuring that AI solutions meet the high standards required by government clients.
The compliance efforts are further complicated by the dynamic nature of the data. Hoopes says a “unified solution” that leverages DSPM and DLP solutions can allow security teams to understand how data is stored, used, and moved across an organization while monitoring its access and keeping pace with ever-changing compliance requirements. Secure Web Gateway (SWG) and Cloud Access Security Broker (CASB) solutions provide further guardrails for teams adopting Gen AI, ensuring that users only access trusted web applications and avoid sharing data with unknown ones.
However, all the above solutions Hoopes talks about have multiple moving parts. One solution the enterprise security expert recommends is a comprehensive GenAI Security Solution that offers visibility, control, and risk-based data protection for businesses and government agencies across GenAI platforms – including integration with OpenAI’s ChatGPT Enterprise Compliance API. The Forcepoint solution merges SSE technologies ( like Forcepoint ONE CASB and Forcepoint ONE Web Security) with information protection capabilities (as those in Forcepoint DSPM and Forcepoint ONE Data Security), creating a framework that safeguards data and intellectual property. Plus, the integration with ChatGPT further supports regulatory compliance and helps enterprises understand how this essential GenAI tool is used throughout the organization.
With the scaling of mega projects, businesses and government agencies face increasing operational complexity. While this technology presents challenges, experts agree that with the right strategies in place—emphasizing security, scalability, and stakeholder engagement—AI will drastically change public services for the better of its citizens.
The success of the Middle East’s ambitious AI adoption plans hinges on several critical factors, including effective pilot programs, comprehensive ethical frameworks, and the establishment of robust infrastructure readiness. Considering much of this is already in motion, it will be interesting to see how quickly businesses, governments, and citizens can adapt to and fully leverage the transformative potential of AI.
Explore innovative tech solutions for efficient, accessible governance at GovTech Conclave in Abu Dhabi on November 21, 2024. The event will spotlight the Middle East’s digital transformation journey and the potential of hyper-automation to revolutionize government operations.