How Human-Informed AI Leads to More Accurate Digital Twins
A hybrid approach that combines human knowledge and machine learning delivers better results for mission-critical simulations.
Topics
News
- Customer Centricity Summit & Awards Explores Brand-Customer Relationships in KSA
- GITEX Global 2024 to Showcase Global Innovation, Investment, and Cybersecurity Trends
- The Perfectly Imperfect Start of Disruptive Innovations
- GovTech Conclave to Explore Cutting-Edge Solutions for Modern Governance
- New Report Shows Cautious Optimism Among Enterprises Adopting AI
- Majority of CISOs Feel Their Organizations are Unprepared for Cybersecurity Regulations
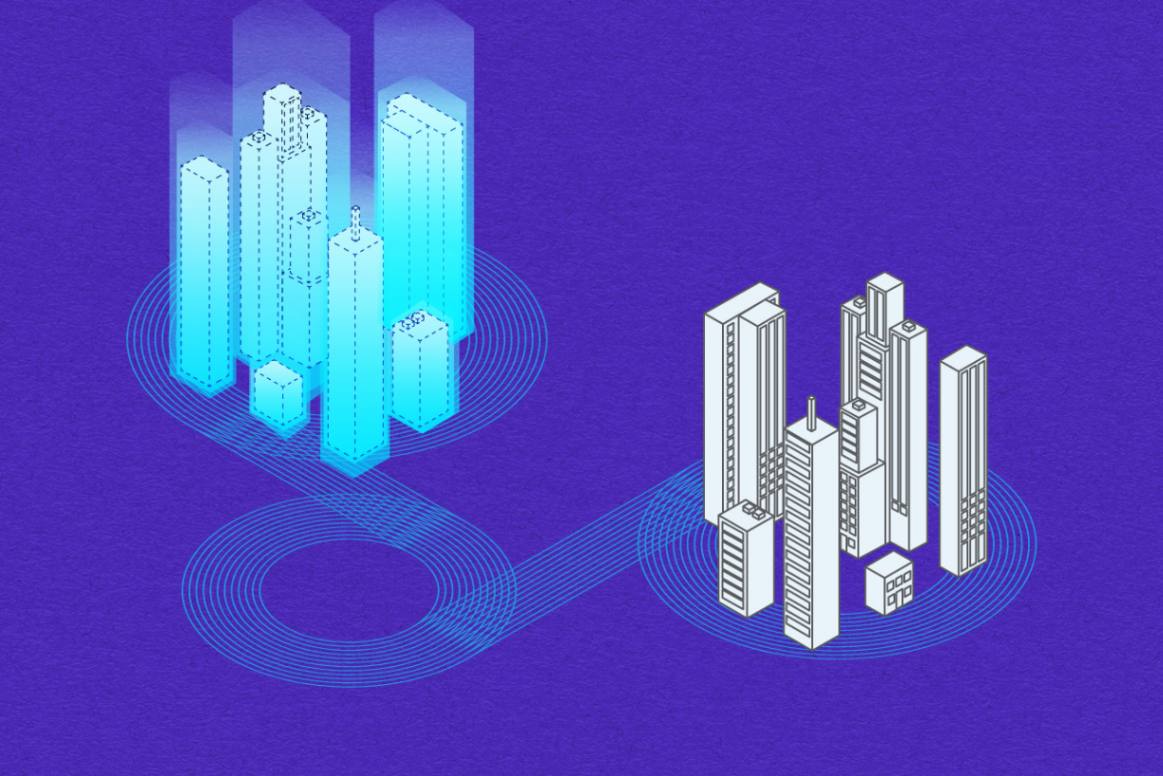
Carolyn Geason-Beissel/MIT SMR | Getty Images
“OK, Houston, we’ve had a problem here,” said astronaut John Swigert in 1970, after an explosion disabled the Apollo 13 spacecraft on its way to the moon, 200,000 miles from Earth. Those iconic words triggered a heroic effort that eventually succeeded in bringing the astronauts home safely. To do so, NASA scientists and engineers needed to develop and test innovative solutions on the fly. A critical tool they used was an Earth-based “twin” of the spacecraft — then, mostly physical — upon which they could experiment swiftly and safely without endangering the astronauts.
Half a century later, this concept has evolved into the digital twin (DT) — a digital replica of a complex real-world entity. DTs comprise two key elements: a high-fidelity model of the entity and a dynamic mechanism to keep the model true in real time, even as the entity undergoes changes. In industrial settings, internet-of-things (IoT) sensors typically provide the data for dynamic updates. DTs are especially powerful tools for mission-critical applications, where experimenting with the physical system isn’t feasible or is expensive, time-consuming, and hazardous.
The value of a DT is directly proportional to its accuracy, which in turn depends on the data available. But data availability remains a challenge — ironically, often in the business use cases that could benefit the most from DTs — and it’s a big reason why DTs are still in their infancy.
DTs could help guide the expansion of current products to new market domains, accelerating R&D and innovation by enabling virtual experimentation. But research activities often involve exploring new territory where data is scarce or protected by patents owned by other organizations. For example, while DTs could inform an organization’s understanding of how a new topology may affect heavy construction equipment or how a smart building may behave under unusual weather conditions, there is limited data available about these new domains.
Based on our experience seeking to apply DTs in data-poor environments, we’ve developed a hybrid methodology that combines human knowledge with artificial intelligence. Such knowledge includes scientific understanding and experiential observations accumulated over decades and even centuries by domain experts.
We begin our approach by using a core of human knowledge to build a preliminary DT model. Next, the optimal AI algorithm is selected and trained with empirical rules and/or a science-based simulator. Then the AI is used to optimize and fine-tune the DT model. The accuracy is further improved with selective IoT sensor data wherever possible. AI excels at data analysis, and it can swiftly blend the empirical, simulated, and observed data to keep the DT current and accurate, even as the actual system changes dynamically. Finally, a human domain expert checks that the AI’s recommended actions are valid and safe. (See “Blending Human Knowledge With AI to Create Accurate Digital Twins.”)
Blending Human Knowledge With AI to Create Accurate Digital Twins
Drawing on human knowledge at the outset when designing digital twins can help supplement sparse data and make it possible to develop an initial model.
How a Hybrid Approach to Digital Twins Works in Practice
We’ve applied the hybrid methodology described above in several different contexts where it could be difficult to obtain adequate data but the case for DTs to add value is strong. Here are three examples from our work in the domains of semiconductor R&D and manufacturing, energy-efficient smart buildings, and construction.
Semiconductor R&D and Manufacturing
In semiconductor manufacturing, the full process to create a complex processor or memory chip has several hundred steps, and each step has dozens of process parameters, such as temperature, pressure, and gas flow rate. The challenge is to maintain each of these parameters within exceedingly tight tolerances and to understand their complex interactions. This often requires expensive trial-and-error experiments and time-consuming analytics. Further compounding this challenge is the business imperative to generate revenue quickly by scaling to high-volume manufacturing.
DTs can add immense value by reducing costs and the time it takes to develop new processes, but data to develop these models is limited given that the work explores new territory. Further, data-sharing across the supply chain is sharply limited due to extreme sensitivity about intellectual property.
Our hybrid methodology offers an effective solution to create an accurate DT. Here, we start with an approximately correct process recipe, developed by experts based on their knowledge and experience. Next, we calibrate a science-based simulator with recipe parameters from human experts. We refine this further with transfer learning from similar domains and past research. An AI model is then trained with simulated data and fine-tuned by sensor data from selected experiments. Advanced data privacy and encryption techniques are used to enable data-sharing between supply chain partners without compromising intellectual property. By integrating all of these elements systematically in the hybrid methodology, we can build DTs that accurately predict chip performance and provide the optimal recipe to achieve the best result.
Energy-Efficient Smart Buildings
DTs can also be used to improve smart buildings, which are designed to improve energy efficiency. Data about the building’s performance in various environments is essential to this undertaking. The challenge is that little data is available about extreme environments. Also, most buildings in the world are older and thus lack sensors and connectivity, further compounding the issue.
In this scenario, we start with a thermodynamics-based physical model of the heating or cooling equipment, then fine-tune it with AI, calibrated with data from selected sensors strategically located in the building. For older buildings, we have developed a unique, portable, and easily deployable sensor platform called building-in-billfold that enables the swift collection of just the essential sensor data to accelerate early learning. The thermodynamics-based model guides us on where the gaps are and what data is essential to collect.
By systematically integrating all of these elements, we create a DT that predicts the building’s behavior accurately. We have applied it to reduce energy consumption, optimize building controls, detect malfunctions, and perform preventive maintenance. Further, our DT can interpolate between sparse data points and extrapolate the behavior of a system beyond the boundaries of the training data set. Thus, we can even use it in novel environments, like under extreme weather conditions or where new heating/cooling equipment is being integrated into a legacy structure.
Construction
DTs can also improve the efficiency and safety of heavy construction equipment by adding intelligent operator-assist features. When a machine is excavating at a new site, there is little data available about exactly what it is digging into. Inaccurate assumptions can lead to increased costs, energy consumption, and safety risks. For example, if the applied force for the excavation bucket were miscalculated, the equipment could be severely damaged or, worse, the operator could be injured.
For this example, we begin with a model based on physics and chemistry, with the best available knowledge of the soil composition at the target location — the size/distribution of rocks and particles, and water content. This model is incorporated into a simulator and calibrated by expert operators familiar with similar terrains. Then we run selected, controlled experiments to observe the soil with cameras and lidar and to measure the impact on equipment parts with stress/strain gauges. Next, AI is harnessed to develop an accurate DT by fine-tuning the physical model with selective experimental data. After a final vetting by human experts, this DT can be used to enhance the safety and efficiency of equipment operating at the target location.
The DTs described above, which we were able to develop with our hybrid methodology, highlight the benefits that this technology can deliver. In semiconductor operations, for example, DTs can reduce new process development times by half. The inclusion of $285 million in the federal CHIPS and Science Act to fund a digital twin institute focused on semiconductor design should accelerate their use.
DTs can substantially move the needle on sustainability metrics in construction, particularly for smart buildings. Our initial results show the potential to reduce energy consumption by 25% to 50%. Again, successful scaling would impact sustainability significantly since buildings consume approximately 40% of the world’s energy and 70% of the world’s electricity, and construction is an $8 trillion industry that generates about 40% of the world’s carbon emissions.
Our experience shows that limited data availability needn’t limit the application of DTs. The results we obtained using our hybrid method to develop three mission-critical applications are preliminary, based on simulations and proof-of-concept pilot experiments. If scaled successfully, such an approach could make it more feasible to use DTs to address a broader array of problems.