MBZUAI unveils next-gen computer vision models for AI in remote sensing
Mohamed bin Zayed University of Artificial Intelligence recently launched five models—BiMediX, PALO, GLaMM, GeoChat, and MobiLLaMA—a major milestone for the institute.
News
- Customer Centricity Summit & Awards Explores Brand-Customer Relationships in KSA
- GITEX Global 2024 to Showcase Global Innovation, Investment, and Cybersecurity Trends
- The Perfectly Imperfect Start of Disruptive Innovations
- GovTech Conclave to Explore Cutting-Edge Solutions for Modern Governance
- New Report Shows Cautious Optimism Among Enterprises Adopting AI
- Majority of CISOs Feel Their Organizations are Unprepared for Cybersecurity Regulations
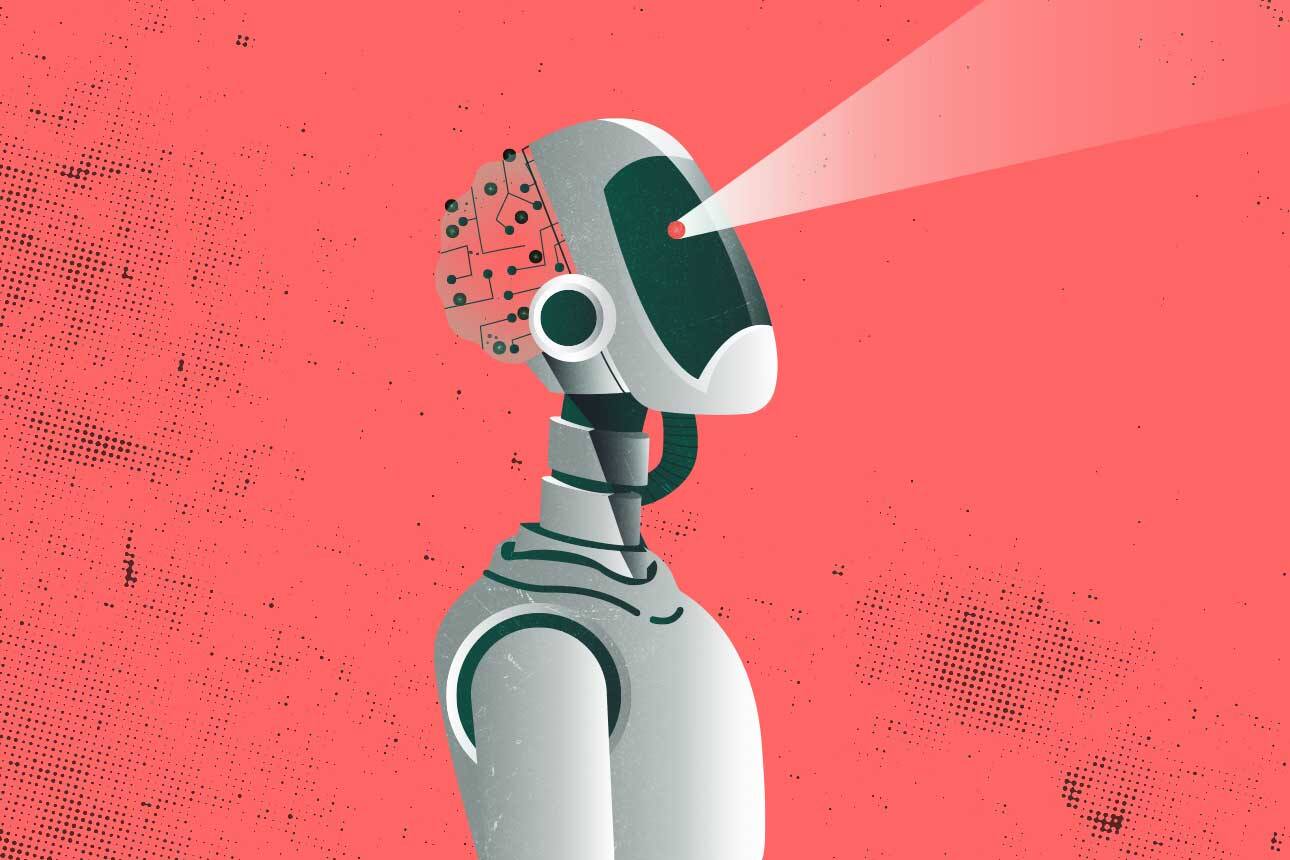
[Image source: Krishna Prasad/MITSMR Middle East]
Since its inception in the 1960s, computer vision has evolved from rudimentary edge detection and shape recognition to nowbeing capable of understanding visual data with unprecedented precision. Early breakthroughs like neural networks and feature-based methods laid the groundwork, but it was the deep learning revolution of the 2010s—particularly through convolutional neural networks (CNNs)—that accelerated its real-world applications. Today, computer vision powers advancements across industries, enabling machines to interpret complex visual information with speed and accuracy that often surpasses human capabilities.
The Mohamed bin Zayed University of Artificial Intelligence (MBZUAI) in Abu Dhabi, United Arab Emirates, is making significant advancements in computer vision research. At GITEX Global 2024, the university presented its latest developments, demonstrating AI’s potential to enhance efficiency and open new opportunities in various sectors.
Dr. Salman Khan, Associate Professor at MBZUAI, delved into the intricacies of computer vision and its significant role within the broader AI ecosystem.
Computer Vision and Geochat
Khan explains that artificial intelligence focuses on developing agents capable of performing specific tasks intelligently across various scenarios. Computer vision plays a key role in any situation involving interaction with visual data—such as images, videos, earth observation data, medical scans, or surveillance data.
“Computer vision is all about understanding the vast visual data we encounter in our world and automating its analysis with machines,” he says. “That is the core goal of computer vision.”
The university launched five new models—BiMediX, PALO, GLaMM, GeoChat, and MobiLLaMA—marking a significant milestone for the institute and the broader AI research community. These models span a range of small to large language and multimodal applications, addressing key areas such as healthcare, visual reasoning, multilingual multimodal capabilities, geospatial analysis, and mobile efficiency, with emphasis on Arabic language support.
GeoChat is the first grounded Large Vision Language Model, tailored to Remote Sensing (RS) scenarios. Unlike general-domain models. It allows for the analysis of high-resolution RS imagery using region-level reasoning, facilitating in-depth scene interpretation.
“We now have access to unprecedented volumes of geospatial data, yet manual analysis is time-intensive and laborious,” says Khan. “Our aim is to leverage AI for automated interpretation and analysis of satellite imagery.”
The model utilizes the conversational interface of generative AI to manage and analyze the extensive satellite data available from Earth observation platforms, unlocking a range of downstream applications through automated analysis. “In the GeoChat extension framework, we are targeting over 38 downstream applications and evaluating the model across this range. It’s trained on a robust visual and language instruction corpus, with up to 22 million instructions.”
The targeted applications include tree species classification, methane monitoring, urban heat island mitigation, and infrastructure monitoring. The model also addresses specific remote sensing challenges, such as ship and vehicle detection and disaster-related change monitoring.
Data Augmentation and Transfer Learning
Khan further adds that they are building on the strong vision and language backbones for GeoChat, leveraging transfer learning to adapt powerful models designed initially for natural images to geospatial applications. The team uses a 4-billion-parameter model, intentionally selected for its lightweight architecture to promote sustainability by reducing resource consumption.
This 4-billion model includes a 3.8-billion large language model trained on text in English and other languages, while the vision backbone has around 340 million parameters. The backbone undergoes a two-stage training process on natural images, then fine-tuned and transferred for geospatial intelligence applications.
Among the main challenges, Khan highlights the need for a data pipeline capable of processing millions of images from various sensors and timestamps. Ensuring high-quality annotations was also crucial, as model accuracy heavily depends on data quality.
Khan further explains the significance of grounded large language models (LLMs) in advancing computer vision for remote sensing. Grounded LLMs generate responses based on visual data, linking language output to specific visual references. For instance, when prompted to locate a red car in an image, the model can provide both a language-based description and the pixel-accurate location of the object.
“These grounded LLMs are very useful for several downstream applications if you want to identify and locate certain reference expressions or objects with certain possible key attributes,” he adds.
The Future of Computer Vision
Looking ahead, Khan states that the university aims to equip its models with human-like visual understanding capabilities, a goal he believes is well within reach at MBZUAI. “The university is truly a unique place right now, as many of these AI sub-disciplines coexist here in one space, giving it a unique position as the world’s only university dedicated solely to artificial intelligence.”
Khan adds that these specialized departments in areas like natural language processing, computer science, robotics, and machine learning enable researchers to collaborate actively across disciplines. This collaborative setting allows them to achieve outcomes that would be challenging to realize independently.