Scaling Automation: Two Proven Paths to Success
Lessons from two leading hospital systems show how to overcome the obstacles to automation.
News
- Data Analysts in UAE and Saudi Arabia Embrace AI-Driven Transformation
- CEOs Recognize AI’s Potential but Fear Knowledge Gaps, Study Finds
- Core42 and Qualcomm Unveil AI Playground to Accelerate Adoption in UAE
- Customer Centricity Summit & Awards Explores Brand-Customer Relationships in KSA
- GITEX Global 2024 to Showcase Global Innovation, Investment, and Cybersecurity Trends
- The Perfectly Imperfect Start of Disruptive Innovations
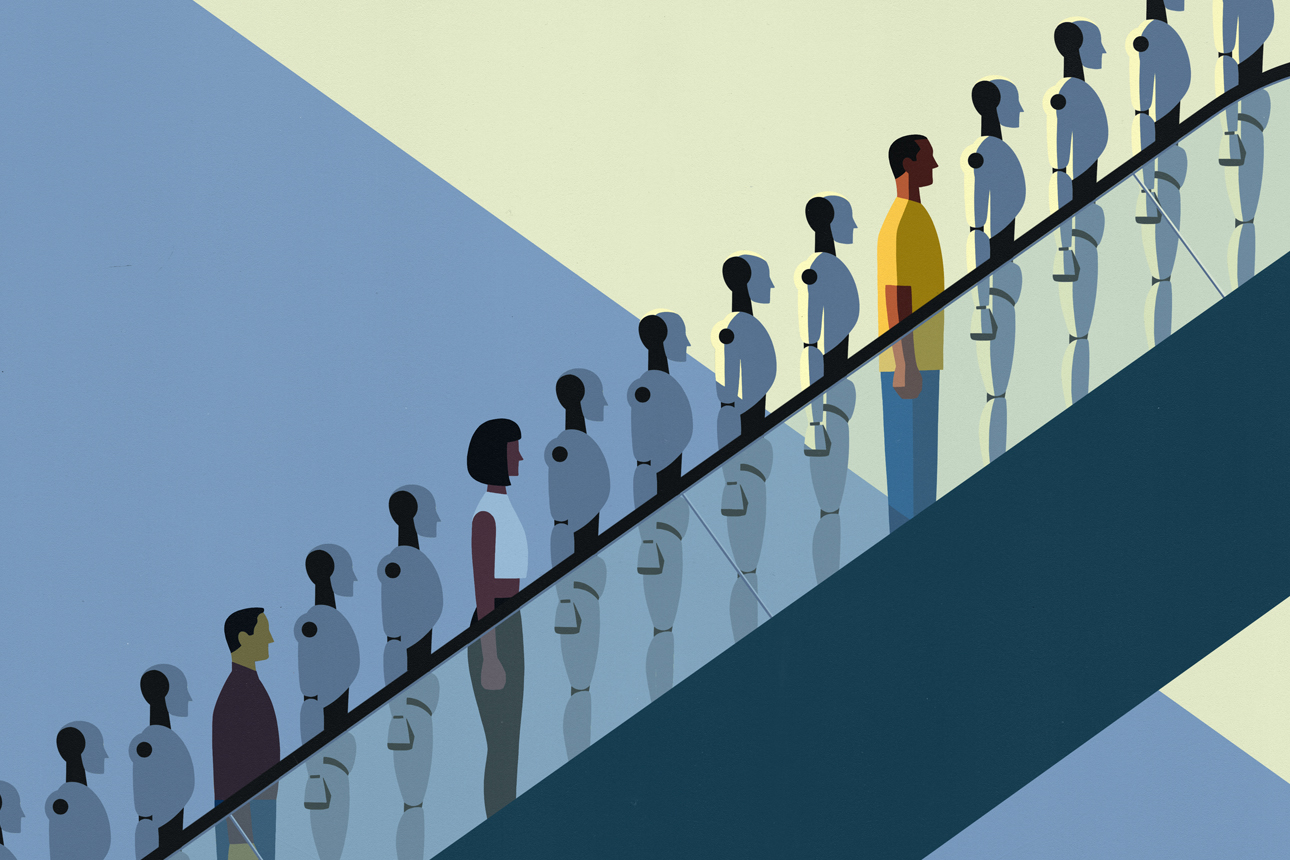
Jon Krause/theispot.com
Organizations faced with a large volume of repetitive manual processes often look to automation to free up their employees to work on more productive tasks. The challenge, however, is deciding how to implement automation in a way that best suits the organization. Which processes should be prioritized for automation? And should the effort be led by technical experts or process experts? A close look at how two hospital systems adopted automation can provide clues to what approaches might work best for other organizations.
In 2018, the finance department of the Mass General Brigham hospital system in Boston was facing a worsening bottleneck in keeping track of the providers in its network. Front-line employees needed to gather up-to-date information on an increasing number of health care providers, but the process was slow and inefficient, requiring three separate hospital administrators to manually collect, aggregate, and export data through a mind-numbing series of clicks.
To help automate this and future processes, the hospital system established a new automation team by recruiting developers to build and manage automation tools and a process specialist from its finance department. The specialist worked with the finance department to restructure its workflow in a way that would lend itself to automation and with the automation team to build a tool that would fit into the new workflow. The tool they developed collected data on providers automatically and organized the information in a way that highlighted the actions the finance team would need to take to move the process forward. This liberated front-line finance employees to do higher-value work and allowed for scalability to manage the expected growth in the number of health care providers in the system.
Two hundred miles away, at Mount Sinai Health System in New York City, a similar push to automate administrative work unfolded differently. The finance team wanted to improve the efficiency of handling patient financial claims and asked Mount Sinai’s IT department to identify processes that could be a good fit for automation.
The IT team identified the process of reviewing claims linked to a big insurance provider as an area where technology could increase efficiency. There was a large volume of work, and the task matched what the organization’s automation technology could do well: follow a set of predefined instructions through an ordered series of tasks. Compared with Mass General Brigham’s, Mount Sinai’s automation effort involved less reengineering of existing workflows. The software engineers could program a bot to handle each claim using the existing process, freeing administrators to oversee only the automated system and troubleshoot exceptions.
Over the past five years, Mass General Brigham and Mount Sinai have developed more than a hundred automations across multiple administrative and clinical departments. The implementation of new technologies has saved tens of thousands of labor hours combined, resulting in tens of millions of dollars in indirect savings — all without leading to layoffs at either organization but instead refocusing employees on activities and projects with higher added value.
Today, many organizations are trying to determine how best to deploy the latest AI software tools at scale. They are encountering many of the same questions that have challenged Mass General Brigham and Mount Sinai: How can teams identify the right tasks to automate or augment with technology? How can deploying new technologies assemble the right combination of process and technical expertise?
A key reason why Mass General Brigham and Mount Sinai have successfully scaled up automation while many organizations have struggled is that they have built internal processes to identify tasks that are the right fit for automation, and they’ve assembled the right knowledge to integrate the technology to perform those tasks.
Successfully scaling automations in large organizations, as these hospitals did, is more often the exception than the norm. In a survey of more than 400 executives across industries, 73% reported using automation software like the tools deployed at Mass General Brigham and Mount Sinai, but only 13% of respondents indicated that they had scaled their use of those tools.1
Managers faced with the question of how to implement and scale automation technologies can learn from the models at Mass General Brigham and Mount Sinai. Despite the organizations’ different approaches, these cases offer shared lessons that help explain why automation at scale has proved so challenging — and how to overcome the obstacles that have stymied their peers.
Finding the Right Automation Fit
When Mass General Brigham and Mount Sinai began exploring how they could introduce automation, it was not obvious which business tasks were the best candidates. Two facts were clear. Robotic process automation (RPA) tools were best at completing routine tasks with clear rules. The more often the organization had to do those tasks — and the longer the tasks took to complete — the greater the potential savings in labor hours that automating the tasks could offer. While this was a good criterion by which to rank tasks for automation, other factors complicated the picture. Routine tasks that seemed like a good fit might have unstructured data that required human judgment for interpretation. Or there might be complex compliance requirements associated with the process that would be hard for a bot to follow.
Both Mass General Brigham and Mount Sinai independently developed well-defined scorecards for evaluating whether a task was a good fit for automation — and how to measure its impact. The scorecards work similarly at each hospital system: A business team identifies a process that seems like a good candidate for automation, fills out the scorecard summarizing the costs and benefits, and sends it to a steering committee of executives for approval.
The scorecards capture two dimensions of the task to be automated: how challenging it would be to automate it (complexity) and the potential value it could provide to the organization (usefulness).2 At Mass General Brigham, business team members responsible for managing complex processes, in partnership with the intelligent automation team responsible for the technology, fill out the scorecard and rate tasks on a scale of 1 to 5, based on 11 factors, to determine their suitability for automation and the level of effort that would be required. Considerations include how structured the data is, how standardized the procedures are, how many applications would rely on the automation, and how much human judgment is required to do the task well.
The scorecard is a tool to measure ROI. It requires an estimate of potential labor savings, which is often the focus of organizations’ ROI calculations when it comes to automation. It also prompts business teams to uncover added benefits to automation outside of the traditional labor-saving metrics. Such opportunities include new potential sources of revenue or revenue acceleration, higher employee satisfaction, increased speed to production, improvement in the quality of work (that is, a reduction of errors), elimination of outsourced work, or opportunities to mitigate risk or fines.
As both hospitals began reviewing dozens of ideas for automation, the scorecards proved beneficial in three key ways.
First, they define the core goals that the organizations are trying to achieve by introducing automation — and prompt business teams to think about their automation ideas in those terms.
Second, the scorecards help democratize the automation process. Rather than having technical experts responsible for identifying automation opportunities, the structure of the scorecards allows front-line workers to consider whether their everyday tasks might be good candidates for automation — and improve their productivity at work.
And third, the scorecard sets quantitative goals in terms of hours saved for each automation project that the teams can compare the actual savings against.
Sample Automation Scorecard
Below is an example of the kind of scorecard used to rate opportunities for automation across multiple dimensions on a scale from 1 to 5 (low to high impact or opportunity), or with actual figures where appropriate (hard savings, hours, etc.). Leaders review scorecards to identify tasks that are good candidates for automation. Different organizations will weigh the various factors differently.
With multiple departments competing for limited resources to build automation tools, Mass General Brigham and Mount Sinai have found it challenging to prioritize which automations to build. The scorecard approach allows automation steering committees composed of hospital leadership to compare and prioritize automation opportunities. Business and clinical leaders can review the scorecards and weigh the strategic value of each opportunity, while IT leaders are able to weigh in on the impact these opportunities might have on their teams and other projects.
In most cases, automation at Mass General Brigham and Mount Sinai has improved the efficiency of processes without displacing workers. Even when the organizations adopt new technologies with the goal of reducing labor and direct costs, most jobs are at best only partially automatable, and the workers in those roles shift their positions to take on work that often adds more value. Mass General Brigham has been intentional about minimizing the negative impact on workers, and a representative from HR has participated in the automation discussions to anticipate the downstream impact on front-line workers and ensure that any worker capacity opened up by automation can be filled by work that adds value. This process is closer to what we consider positive-sum automation, which boosts productivity for organizations while increasing flexibility for employees.3
Embedding Technical Knowledge on Front-Line Business Teams
To implement automation technologies, organizations need technical knowledge to customize and operate the technology tools as well as process knowledge to identify the highest-impact processes that are good candidates for automation. Combining technical and process knowledge is critical to the successful deployment of automation. The most successful cases of technology implementation restructure work processes as new technologies are deployed.4
The problem is that technical and process knowledge often reside in different parts of an organization. Technical knowledge is concentrated in IT departments and on engineering teams, whereas process knowledge can be distributed among subject-matter experts on front-line teams performing administrative tasks that are candidates for automation.
Mass General Brigham and Mount Sinai represent different ways of solving this problem. Mass General Brigham led with process knowledge when creating the role of the automation developer, who was charged with programming RPA software to perform administrative tasks. Automation developers at Mass General Brigham typically had experience in how the hospital system’s processes worked but only limited technical knowledge. They were trained to use RPA tools to program software bots.
Paths to Implementing Automation
The two hospital systems took different paths to implementing automation at scale. Mass General Brigham armed its process experts with technical knowledge, and Mount Sinai equipped its technical experts with process knowledge.
One automation developer at Mass General Brigham classified their role as process improvement specialist. Even though they are technically programming software, they said their first job is identifying how a process could be fixed and then picking the technology most appropriate for fixing it. Mass General Brigham’s approach to combining process and technical knowledge is to arm process experts with technical knowledge.
For example, Mass General Brigham had a frustratingly fragmented process for checking that nurses’ and medical technicians’ professional credentials were up to date across the organization. Automation developers had to document and standardize the credential verification process across the hospital system before tackling the technical challenge of building a bot that could automate it. The resulting automation allows for consistent staffing plans across the hospital system without unanticipated shortages due to expired credentials.
The trade-off of the Mass General Brigham approach, however, is that its process experts have limited technical knowledge. Its automation developers might not be able to use machine learning tools to improve a process, since they are only trained to work with RPA software. The hospital system’s former CFO said the organization’s goal is to excel at fixing bad processes so it can then incorporate more sophisticated tools that use optical character recognition (OCR), artificial intelligence, and related technologies.
Mount Sinai took the inverse approach, arming technical experts with process knowledge. The hospital system recruited experienced software developers to its IT team who scan the organization for processes that their technical capabilities could automate and make more efficient. Once an area is identified, the IT team will then study how subject-matter experts did their jobs and map complex processes before trying to automate them.
Having a team of full-stack developers on the IT team has enabled Mount Sinai to automate more processes by building more complex technology applications. For example, the developers created an OCR tool to automate the processing of patient consent forms filled out by hand with much greater accuracy than the previous software had been able to, reducing the need for administrators to manually double-check forms.
The benefit of the Mount Sinai approach is the capability to extend new technologies to automate more complex processes. The risk of this approach is that the technical experts are limited by the amount of process knowledge they are able to assemble. Even if the technical experts conduct rigorous interviews and process-mapping exercises with the various business teams, they will inevitably miss some of the tacit knowledge that the process experts have acquired over years of experience. The challenge with the tacit knowledge is that it cannot readily be codified; process experts “can know more than we can tell,” as the sociologist Michael Polanyi wrote.
Mass General Brigham and Mount Sinai were able to succeed with their automation projects by embedding technical experts on process-focused teams or process experts on technical teams. By adopting this approach, teams pursuing automation projects began to develop a shared language about how the process works and where technology could be deployed to make an impact.
The two hospital systems built an automation capability with two key dimensions. One is a common scorecard for evaluating and measuring the impact of automation projects using various technologies, including RPA, OCR, and AI. The other is an organization that assembles the technical and the process knowledge both to fill out the scorecard and to implement the automation projects that receive investment from the organization.
Scorecards are important because they provide front-line workers with a channel to turn their ideas into action — and a way for managers to put guardrails on the idea-generation process. Combining diverse sets of knowledge matters for automation implementations because process experts can identify the gaps that technical experts can’t see. As in the employee licensing example, the process experts needed to identify how the process should operate before technical experts could come in and program a bot to automate parts of it.
Mass General Brigham’s approach highlights how empowering front-line workers to identify processes that are a good fit for automation — and connecting them with technical skills — can expand and accelerate the potential set of processes that an organization can automate. Mass General Brigham’s deliberate engagement of workers with process knowledge offers a lesson for Mount Sinai and other organizations that have adopted an IT-led approach.
The Process-Technology Trade-Off
Organizations can achieve more from automation when they combine process and technical knowledge to implement new technology tools.
The lesson from Mount Sinai, on the other hand, is how developing internal technical knowledge of a variety of automation tools can accelerate an organization’s ability to address more complex problems. Even when organizations like Mass General Brigham and Mount Sinai rely primarily on software from third-party vendors that’s advertised as low-code or no-code, it turns out that internal technical knowledge proves critical for identifying what is the best fit for automation.
References
1. J. Watson, G. Schaefer, D. Wright, et al., “Automation With Intelligence: Pursuing Organisation-wide Reimagination,” PDF file (Deloitte Insights, 2020), www2.deloitte.com.
2. The scorecards reflect a long-standing framework for evaluating “technology acceptance.” See F.D. Davis, “Perceived Usefulness, Perceived Ease of Use, and User Acceptance of Information Technology,” MIS Quarterly 13, no. 3 (September 1989): 319-340.
3. B. Armstrong and J. Shah, “A Smarter Strategy for Using Robots,” Harvard Business Review 101, no. 2 (March-April 2023): 36-42.
4. J.P. MacDuffie and J.F. Krafcik, “Integrating Technology and Human Resources for High-Performance Manufacturing: Evidence From the International Auto Industry,” in “Transforming Organizations,” eds. T.A. Kochan and M. Useem (New York: Oxford University Press, 1992), 209-225; and E. Brynjolfsson and L.M. Hitt, “Beyond Computation: Information Technology, Organizational Transformation and Business Performance,” The Journal of Economic Perspectives 14, no. 4 (Fall 2000): 23-48.