The Enduring Power of Data Storytelling in the Generative AI Era
Conversations with tools like ChatGPT work great for some decision-making situations, but not all. Here’s how to best deliver data for four key cases.
News
- Businesses Face Significant AI Adoption Gap, Survey Finds
- Gartner Identifies Key Data and Analytics Trends Shaping 2025
- GovTech Conclave 2025: Shaping the Future of Digital Governance in the Middle East
- UAE to employ AI to combat and penalize consumer fraud
- Amazon Web Services Ocelot Chip Aims to Cut Quantum Computing Costs by 90%
- VAST Data Unveils New Capabilities to Strengthen AI Data Platform
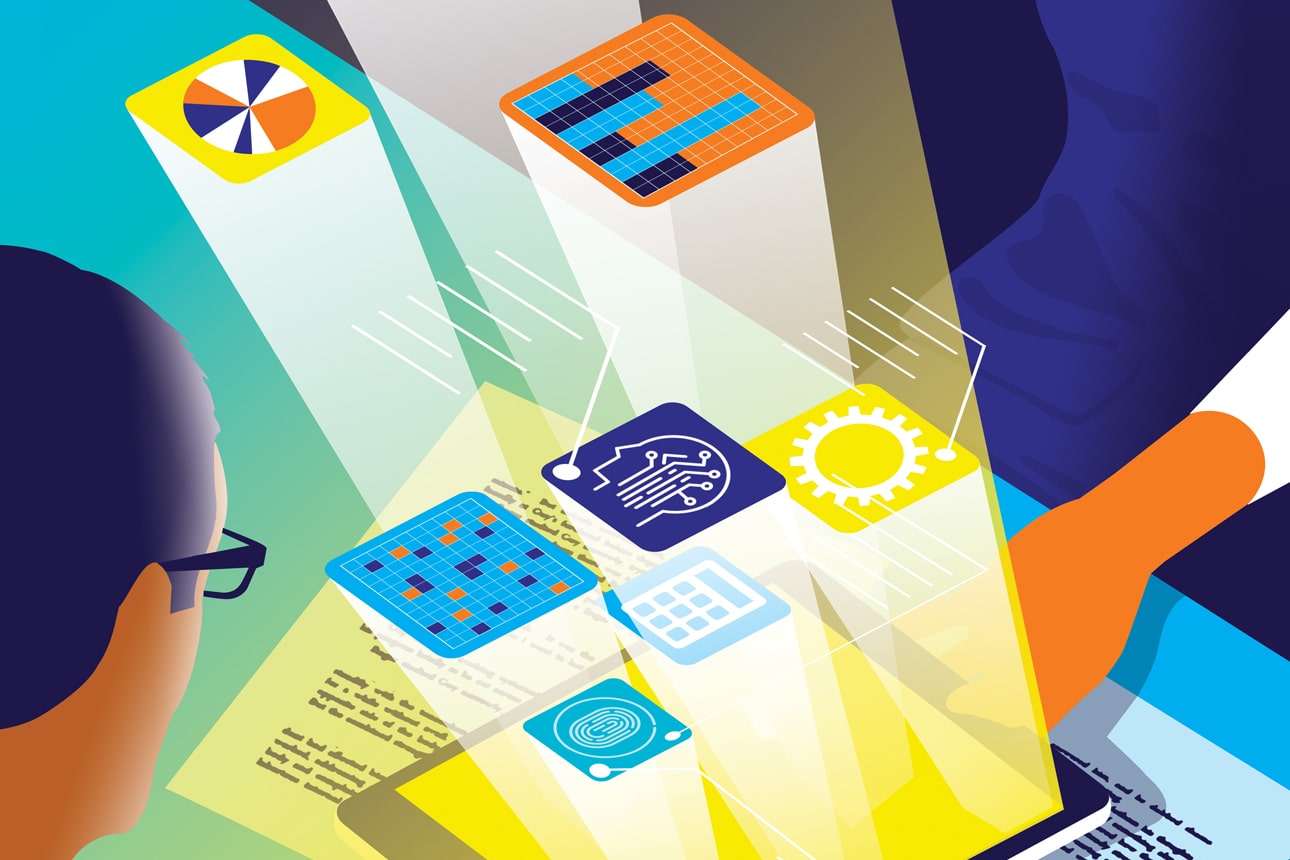
Neil Webb/theispot.com
Today, generative artificial intelligence tools like ChatGPT and Gemini perform an impressive set of tasks with just a few simple prompts. They interpret even poorly formed user questions, identify deep domain insights from data, and share answers as well-structured recommendations. Increasingly, these exchanges are getting conversational, using text-to-speech AI technology. AI can answer questions off the bat, like a (true) executive assistant. Not surprisingly, that has led people to question whether the visual presentation of information is relevant anymore — and even to sound a death knell for visualization dashboards and data stories. Not so fast. Yes, the latest advances in generative AI can help people automatically discover hidden patterns from numbers and unstructured data such as text, audio, or video. It is now possible to translate insights into business recommendations, almost at the level of a domain expert. This eliminates a lot of manual effort and inefficiencies involved in creating and consuming data visualizations. However, trying to entirely do away with the visual display of information is a costly mistake. There is a strong case for deploying conversational intelligence while retaining the magic of visual narratives in certain critical scenarios. Here, I’ll share a simple yet powerful framework to help leaders and their teams understand four key decision-making situations and how to pick the right information presentation tool for the job. We’ll see where AI is ready to fully automate information presentation and where it can play only a secondary role enabling data teams. Today, leaders have the critical responsibility of making these distinctions while deploying AI tools. They must set the tone and address the fears of automation within organizations — for people who analyze and present data and people who make decisions with it.
The Power of Visual Data Narratives
In contrast with tables of numbers, visual narratives use images and charts to communicate complex information quickly and effectively. They engage both the visual and cognitive senses, leading to better comprehension and retention. The visualization dashboard, which rose to prominence in the 2000s, is the most popular information design technique. During the past two decades, enterprises have converted thousands of spreadsheet reports into visual dashboards. These dashboards often have a rich layer of interactivity in the form of dropdowns or clickable elements. This helps users answer questions by exploring insights dynamically, based on specific business challenges. In a hypothetical example, to understand the quarterly performance of salesperson Tom Jones in the Boston area for consumer laptops, a sales leader could filter the interactive dashboard for “sales manager,” “region,” and “product.” Over the past decade, insights delivered through data stories have become prominent in enterprises. While a data story is often confused with a dashboard, it has an important distinction. As the name implies, data stories have a tailored narrative presented as visual insights, with takeaways to help users act. In the above example, a data story might show that Tom Jones missing the past quarter’s sales targets was unusual, using a trending view. It could spotlight the two products he performed poorly on compared with the rest of the team and suggest what the sales leader could do next. While most dashboards expect the user to explore and find their own insights, data stories come with a preset storyline presented in an engaging manner. As Nobel laureate Daniel Kahneman famously said, “No one ever made a decision because of a number. They need a story.”
The Rise of Conversational Intelligence
A few years ago, AI-driven analytics products that could take inputs in natural language, as opposed to effort-intensive programmatic commands, made it simpler to create dashboards. For example, you could ask, “What’s the sales performance of Tom Jones in Boston for the consumer laptops category?” in natural language to generate a tailored visualization dashboard on the fly. Teams could avoid the painstaking process of collecting user requirements, writing SQL queries to fetch the data, and building visualization dashboards by picking charts and applying design principles. While this was a big shift, it was limited by the accuracy of natural language processing and the challenges in understanding business nuances. Then generative AI burst onto the scene and quickly became mainstream, thanks to AI products that can generate images and text, such as ChatGPT, Dall-E, and Stable Diffusion. Now AI tools like ChatGPT can not only process human language accurately but can also speak back naturally with surprising fluency and finesse. What does this mean for the presentation of information? AI has supercharged the ability of analytics products to understand natural language with incredible accuracy and translate the numbers and statistical outputs into human-understandable form with deep domain context. But while this effectively eliminates the need to see results in situations that call for quick information retrieval, it doesn’t serve every decision-making scenario.
Picking the Right Information Presentation Tool for the Job
Two key factors influence how information must be delivered for effective actions — namely, the data insight approach and the level of information that users need. Let’s look at each in turn. Data insight approach: Should the discovered insights be explained to the user, or do users need the ability to explore their own insights? The answer to this explanatory-versus-exploratory question determines the correct approach to delivering the information. Level of information that users need: What is the level of information and associated context that users need to make decisions? It could be simple and straightforward, like understanding last month’s sales performance. Or it could be far more sophisticated, such as when leaders are making a strategic decision on a big-ticket investment. In the latter case, users need a lot of context about the return on investment, historical performance, market trends, and competition. These two factors are the base of a 2×2 matrix that you and your teams can use for guidance in picking the right information delivery mode.
What Kind of Decision Are You Making? Four Situations
This matrix can help you select the best approach and tool for decision-making by business users. First, ask what kind of decision the user needs to make. Is their question exploratory and simple, as shown in Quadrant 1? AI chat tools work well in this case, providing users with simple answers to a series of dynamic questions about topics like monthly sales. Conversely, is their question explanatory and sophisticated, as shown in Quadrant 4? An example here is addressing questions from board members about the business case for a new product. In this type of situation, people need to tell a nuanced data story that AI tools can’t handle automatically or conversationally. Other examples of real-world data questions and the approach best suited to them are shown below.
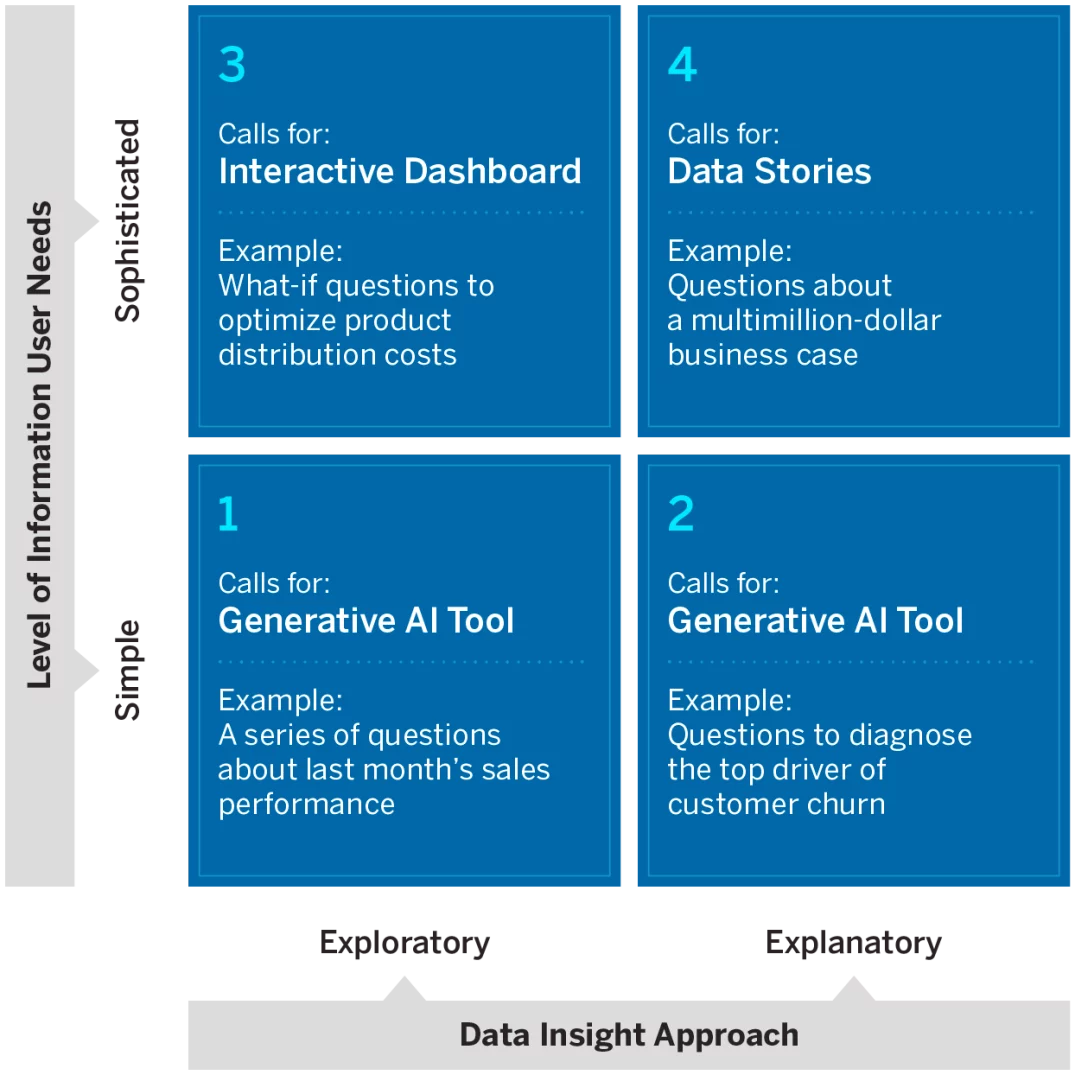
Quadrant 1: Exploratory (Simple)
When users look for simple answers to a series of dynamic questions, the situation falls into this category. The desired answers are often descriptive insights that summarize past performance, such as revenue growth or level of employee satisfaction. Here, the user needs the flexibility to ask questions to explore patterns dynamically. This need has historically been addressed by analysts performing ad hoc analytics and sharing insights through a series of PowerPoint decks or dashboards. This area is ripe for disruption by AI-enabled chat-based interfaces. Imagine enterprise versions of tools such as ChatGPT or Claude that are trained to answer user questions based on organizational data. HR leaders could use these tools as handy analytics assistants to get quick responses to daily queries on employee onboarding, payroll process, or learning and development activities. Given the need for a rapid response to a variety of simple questions, generative AI is a great fit here.Quadrant 2: Explanatory (Simple)
Sometimes a groundbreaking insight needs to be explained to users in order to help them act. For example, when the marketing head grapples with the challenge of customer churn, they might want to understand the top drivers of churn in the past quarter. These descriptive or diagnostic insights demystify why something happened, with likely root causes. Here, the user needs answers to a few predecided, important, and nuanced questions. This need has typically been addressed through statistical analysis and pattern-spotting to uncover hidden insights, usually presented by analysts through decks or dashboards. Today, AI has the ability to understand the business context and discover hidden data insights. These insights can then be presented conversationally via interactive chat to help the user absorb and act. For example, business intelligence (BI) tools such as Power BI or Tableau can be infused with generative AI capabilities to explain insights and interpret takeaways. Early generative AI integrations exist already, such as Copilot for Power BI and Einstein Copilot for Tableau AI. Even better, these insights could be surfaced through conversational interfaces rather than waiting for users to open dashboards and consume them visually. Hence, this is another area ready for disruption by AI chat interfaces. Leaders must understand that data quality needs to be solid for the AI tools to be effective. Data engineering work, involving a lot of human effort, will still be needed to collect, transform, and curate data. Generative AI tools do not equal total automation of all data efforts.Quadrant 3: Exploratory (Sophisticated)
Now let’s get into the more sophisticated scenarios. There are times when users need to explore insights dynamically, and it’s important for people to compare notes across multiple influencing factors or business situations. Say, for example, that the supply chain head of a manufacturing firm is interested in optimizing distribution costs. They might want to understand the key cost drivers and perform what-if simulations to understand how to reduce the delivery cost. In this scenario, they need the tools not only to answer the questions dynamically but to also present a lot of information in a concise, engaging, and understandable manner. Interactive dashboards are a great fit here since they can take advantage of the visual display of information and enable rich interactivity through what-if simulations. Open-source, programmatic data visualization tools, such as Vega or D3.js, can help compose a custom visualization front end with deep interactivity, while what-if scenario modeling can be enabled by analytics tools such as KNIME and H2O. This show-and-tell is crucial to augment the insights and help the supply chain head address their distribution challenge. While AI is getting better at understanding domain knowledge, it is not ready to address this need automatically yet. AI tools can aid in the creation of the analytics engine or enhance the experience of the user interface.Quadrant 4: Explanatory (Sophisticated)
Imagine a board meeting where six key executives have assembled to decide whether to make a big-ticket investment next year. After weeks of analysis, the product head has built a case to commit $50 million to launch a new product. In order to influence the executives to give the go-ahead, the product head needs to present the insights. The information must be presented with many supporting details, such as customer preferences, revenue potential, market opportunity, competitor moves, and reasons why the organization can pull it off successfully. In this situation, the answer clearly needs a lot of context and sophisticated detailing. This is the realm of data storytelling: to inform the audience, influence them with evidence, and inspire speedy action. While AI can assist leaders in assembling some of the information, as seen in the earlier quadrants, it can address this need neither fully automatically nor conversationally — yet. However, the generative AI tools called out above can be useful collaborators for the technology team throughout the process of discovering insights, brainstorming the storyline, choosing pertinent charts, and refining the takeaways. The information can be presented in the form of a deck or through the open-source or enterprise BI visualization tools described above. As the size of the quadrants above indicate, a high number of business challenges fall into quadrants 1 and 2, where AI and conversational intelligence can deliver insights most effectively and efficiently. However, in the realm of quadrants 3 and 4, when the user needs a lot of context and the ability to consume sophisticated information, data stories and interactive dashboards are still the go-to medium.Collaborating With Gen AI to Enhance Data Narratives
As with all things AI, it’s important to remember that pitting AI tools against humans is limiting. There is great power in combining forces. While generative AI is not ready to entirely replace interactive dashboards or the data narratives associated with quadrants 3 and 4, it is already enhancing the data story life cycle in several ways. Data scientists and analysts are using tools such as ChatGPT’s Data Analyst to semiautomatically identify deep analytical insights. Visualization designers are working with Claude AI to cocreate visualizations and automatically create visual design assets. Business subject-matter experts and domain experts are using AI to understand the implications of analytical insights and translate statistical summaries into convincing business recommendations. In 2021, Gartner predicted that data stories will be the most widespread way of consuming analytics by 2025 and that augmented analytics techniques will generate 75% of these stories. With the widespread adoption of generative AI over the past year, it’s quite likely that this number will be much more than 75% and will be realized much earlier. Using generative AI in quadrants 1 and 2 instead of wasting time creating thousands of BI reports or dashboards could save effort and improve business outcomes by increasing the speed of insight delivery. This could also help organizations focus human effort and attention on the more specialized use cases of quadrants 3 and 4, where expert care and the human touch are needed to empower decision makers. Leaders who know how to make those kinds of choices when presenting data will help their organizations survive and thrive in the coming decade.Keen to know how emerging technologies will impact your industry? MIT SMR Middle East will be hosting the second edition of NextTech Summit.