What Machines Can’t Do (Yet) in Real Work Settings
Across the vast range of real-world usage scenarios, there have been far more instances of augmentation of human work by smart machines than of full automation. That scenario is expected to continue for the foreseeable future.
Topics
News
- AI professionals form a redefined workforce. But systemic roadblocks persist, survey finds
- AI-Driven Scams Surge as Microsoft Blocks $4 Billion in Fraud Attempts
- Identity-based Attacks Account for 60% of Leading Cyber Threats, Report Finds
- CERN and Pure Storage Partner to Power Data Innovation in High-Energy Physics
- CyberArk Launches New Machine Identity Security Platform to Protect Cloud Workloads
- Why Cloud Security Is Breaking — And How Leaders Can Fix It
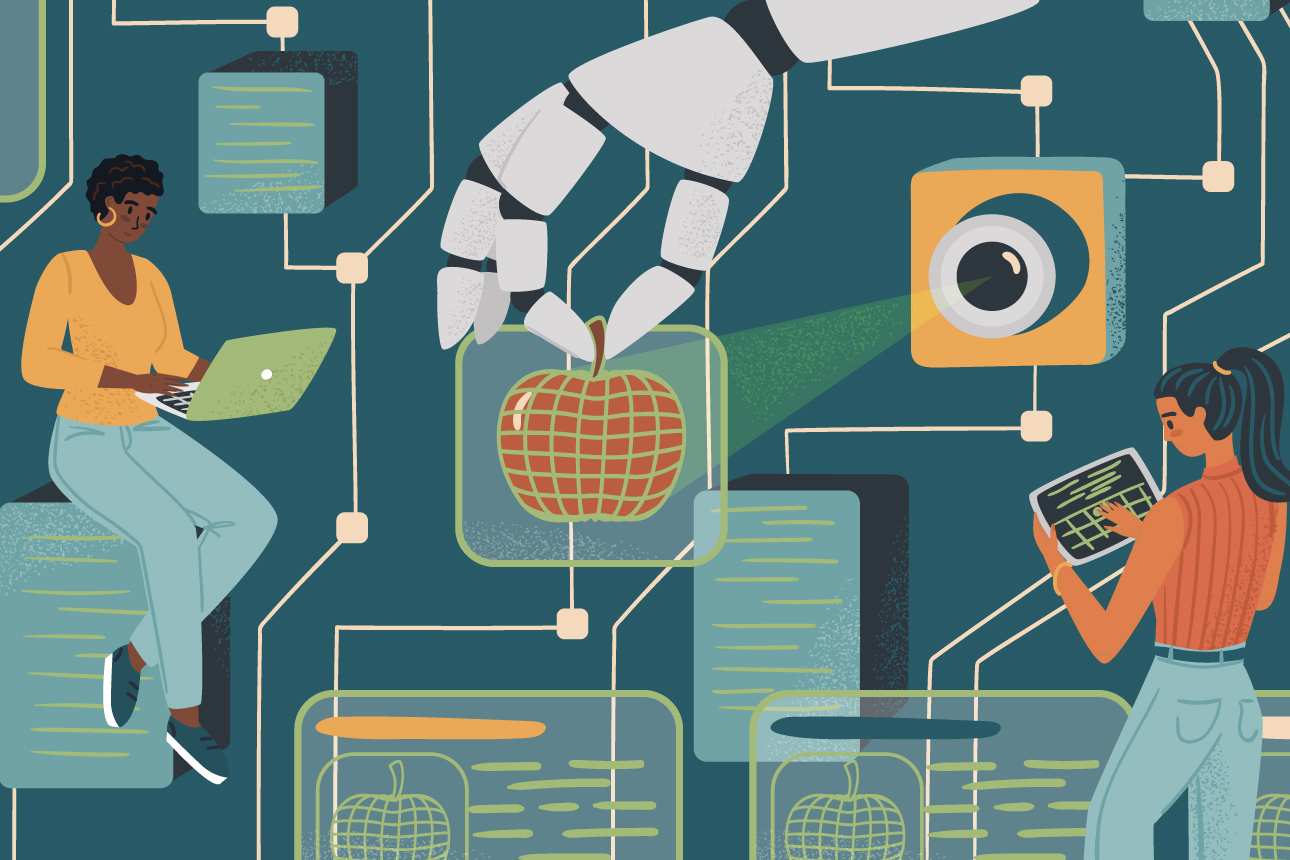
Almost 30 years ago, Bob Thomas, then an MIT professor, published a book called “What Machines Can’t Do.” He was focused on manufacturing technology and argued that it wasn’t yet ready to take over the factory from humans. While recent developments with artificial intelligence have raised the bar considerably since then for what machines can do, there are still many things that they can’t do yet or at least not do well in highly reliable ways.
AI systems may perform well in the research lab or under highly controlled application settings, but they still needed human help in the types of real-world work settings we researched for a new book, Working With AI: Real Stories of Human-Machine Collaboration. Human workers were very much in evidence across our 30 case studies.
In this article, we use those examples to illustrate our list of AI-enabled activities that still require human assistance. These are activities where organizations need to continue to invest in human capital and where practitioners can expect job continuity for the immediate future.
Current Limitations of AI in the Workplace
AI continues to gain capabilities over time, so the question of what machines can and can’t do in real-world work settings is a moving target. Perhaps the reader of this article in 2032 will find it quaintly mistaken about AI’s limitations. For the moment, however, it is important not to expect more of AI than it can deliver. Some of the important current limitations are described below.
Understanding context. AI doesn’t yet understand the broader context in which the business and the task to be performed are taking place. We saw this issue in multiple case studies. It is relevant, for instance, in a “digital life underwriter” job, in which an AI system assesses underwriting risk based on many data elements in an applicant’s medical records but without understanding the situation-specific context. One commonly prescribed drug, for example, reduces nausea for both cancer patients undergoing chemotherapy and pregnant women with morning sickness. As of yet, the machine can’t distinguish between these two situations when assessing the life insurance risk associated with this prescription.
We also saw instances where AI systems couldn’t know the context of the relationship between humans. A fundraising application at a university, for example, selects potential donors and composes email messages to them, but it may not know about a recent death in the donor’s family (which might make it an inappropriate time to ask for a donation) or a recent win in the conference sport’s championship game (which should perhaps be mentioned in the email). Today, there is no good way to represent broad and nuanced context in either mathematical algorithms or rules, and it seems unlikely that this AI deficiency will change anytime soon.
Prioritizing alerts. Computerized systems, sensors, and AI-based image and sound analysis systems create frequent alerts, whether they involve security, health, or machinery issues. In a case study involving security alerts at a large shopping mall connected to Singapore’s Changi Airport, it was clear that a human role was necessary to filter and prioritize the alerts. In complex and constantly changing settings, AI systems are often unable to distinguish between real and important alerts and false or less important ones. Though the AI surveillance systems are very good at prioritizing the most likely alerts for (human) investigation, the assistance of human security guards and system operators is needed to reliably do so.
A similar process and set of challenges took place at Singapore-based DBS Bank, where AI and humans work closely together to prevent money laundering and other types of fraud in its Transaction Surveillance organization. An older-generation rules-based AI system created alerts on suspicious transactions but with many false positives. Even though a newer-generation machine learning AI system prioritizes them, humans still needed to be involved in investigating the alerts.
Weighing subjective elements. One of the managers we interviewed at DBS said there would always be a need for humans in the process because “there will always be a subjective element to evaluations of what is and is not suspicious.” They could include a customer’s extended business, personal, or family relationships, or previous contacts with bank personnel. Perhaps at some point, AI will be able to judge an individual’s honesty and trustworthiness through its analysis of facial images and voice patterns — some financial institutions in China already use this approach. We think it’s more effective to employ humans with subjective judgments to augment AI-data-driven, algorithmic assessments, especially when assessments are heavily influenced by cultural, contextual, and subjective factors. Of course, both humans and machines may be biased in their judgments, and human biases can sometimes be inadvertently trained into a machine learning system. Having humans check on machine decisions and vice versa can be one way to minimize bias.
Analyzing tone. The financial software company Intuit introduced a tool for improving the grammar and language for internally generated content of various types. While the AI system could generate many suggestions for how to improve written text, it couldn’t effectively analyze tone. This issue is also found with AI-based social media analytics, which have had a difficult time understanding sarcasm. Even the most capable natural language generation tools sometimes generate content that is offensive to human readers. It seems likely, then, that humans will need to continue to evaluate content to ensure that it is palatable to sensitive human tastes.
Understanding emotional situations and needs. One AI-human collaboration we observed at Stitch Fix, an online clothing retailer that distinguishes itself by making custom product recommendations, illustrates a key AI shortcoming. An AI system recommends clothing items that customers are likely to buy, but it is unable to take emotional needs related to special occasions into account. Clients are invited to send “request note” comments to their stylists, such as “My husband is returning from being stationed overseas” or “I’m just about to start a new job and need to dress to impress,” and, of course, the AI system doesn’t know what to do with them. Making sense of such comments is one of the reasons why Stitch Fix employs thousands of human stylists to work in partnership with the AI recommendation tool. Understanding emotional situations may be among the last things that AI learns how to do, if it ever does. Humans seem safe in this role for the foreseeable future.
Evaluating and choosing the very best option. AI is good at taking an initial stab at a decision, but when that decision is consequential, humans often need to weigh in and make a final judgment. We observed this situation at Morgan Stanley, where a “next best action” system using machine learning identifies personalized investment opportunities for clients and suggests them to the company’s financial advisers, who then decide which ones to send to the clients.
The “best option” issue rises to another level when life-or-death questions arise — as in health care situations in which humans and AI work closely to diagnose and treat disease. For instance, the Good Doctor intelligent telemedicine system in China and Southeast Asia uses AI to present triage and treatment recommendations to hundreds of millions of patients. Similarly, we saw a dermatology imaging application where AI helped in capturing and presenting relevant images to the dermatologist. In both cases, however, the physician made the final decision on diagnosis and treatment.
This modesty of ambition, particularly in medicine, is an important attribute of AI deployment success. While someday AI may be able to make comprehensive and accurate diagnoses and treatment suggestions directly to patients, today its powers are limited.
Framing problems, and then training and coaching. It is almost miraculous to us that AI can now automatically generate machine learning models, as in the case we researched at the supermarket chain Kroger and its data science subsidiary 84.51°. Automating some data science tasks is an ironic affront to highly intelligent data scientists, and some of the experienced data scientists at 84.51° were initially concerned that they would be moving to a world in which their hard-earned knowledge of algorithms and methods would have no currency. However, the scientists at Kroger were relieved to find that AI can’t frame the problem to be solved in the first place or find data to address that problem. Only humans can identify decisions that need to be made and identify data sets that might shed light on them.
The data scientists at 84.51° also spent considerable time training, coaching, and reviewing the work of less experienced amateurs who use automated machine learning capabilities. We saw similar scenarios at many other companies as well, and we believe the jobs of most data scientists will thus be safe for a while.
Creating new knowledge and transferring it to a system. We discovered an implementation of AI-assisted augmented reality to be used for employee training at a U.S. manufacturing company called PBC Linear. That case illustrates the need for humans to create new knowledge before it can be transferred to an AI system: Experienced machinists were needed to create training materials that could then be consumed by novices using a learning management system and an augmented-reality headset. The system works well but only because it was created by a human in the first place. AI won’t be able to extract and appropriately organize work-relevant knowledge from experienced human brains anytime soon.
Orchestrating physical settings for analysis. AI can conduct analyses of physical or chemical entities, but that can’t happen without a human to set up the analysis process and situation. We found two examples — an AI-based predictive maintenance application for diesel locomotive oil at Massachusetts’s transit authority (the MBTA) and an AI system for automated visual inspection of disk drive head circuitry at the data storage company Seagate — in which an algorithm was effective in analyzing data and recommending appropriate action. However, neither analysis could happen without humans defining, gathering, and structuring the data; designing and setting up the apparatus; and monitoring whether the process is working appropriately. AI is excellent at solving problems that humans have structured for it, but it doesn’t know where to start by itself.
Understanding complex, integrated entities. It’s clear that AI can provide useful predictions and insights about complex, interconnected entities. We found that capability in our Singapore Land Transport Authority case, where a government agency monitors and detects anomalies in a complex urban rail transportation network for the city-state. However, the insights were not reliable or accurate enough to eliminate the need for human staff members at the centralized operations monitoring center. As the managers in that case put it, the AI and related systems notify the staff of transit issues, and “this gives us the ability to make informed decisions on how to deal with the problem.” The transit network is too complex to turn it all over to even the best AI.
Exercising discretion about when to use AI. Even when AI is technically capable of performing a work task, it might not be good at it. We studied the use of Flippy, a hamburger-flipping robot used in several hamburger chains. A franchise manager at one site decided that Flippy was good at frying but was not sufficiently accomplished at flipping burgers to be put to work on that job. We doubt that Flippy was aware of its own shortcomings, and that will generally be true of AI. Only humans, then, can decide whether AI should be used in a particular application.
Considering ethical implications. Companies are beginning to realize that AI systems can have important implications for organizations, employees, and society. AI can’t consider and address these implications, but humans can. Thus far, the leaders in taking action on ethical issues in the commercial sector are software companies, perhaps because their industry has the most potential ethical problems.
The Salesforce ethical AI practice case we researched provides a good illustration of what humans can do. The members of that group evangelize inside and outside the organization, coach, develop guidelines and tactics to address ethics, and help to identify and implement frameworks and tools to support this effort. These tasks are unlikely to be taken over by AI itself in our lifetimes.
A case involving the use of AI-based gunshot detection technology and patrolling recommendations at the Wilmington Police Department in North Carolina had the potential for problematic ethical issues. Humans at ShotSpotter, the vendor for both of these applications, took many steps to address and mitigate such problems and designed them into their software. For example, for the patrolling recommendations, the system does not use any personally identifiable information for crime forecasting, and no individual citizens are ever identified by the system. Alarms on gunshot detection are sent only to the ShotSpotter command center and are reviewed by human experts to verify true gunshots versus other possible causes. Only after this vetting are alarms sent to the police. Of course, the police have to exercise great care in the way they make use of the data on gunshot detection so as not to inappropriately use it as evidence. This is another dimension of ethical consideration that requires human judgment.
Correcting its own errors. In a “digital weeder” case that we studied, an AI image analysis system contained within a piece of robotic farm equipment distinguished between weeds and a farmer’s lettuce crops and then automatically cut down the weeds. But a human helper walks behind the weeding system, looking for signs of mechanical or software-based trouble. If something goes wrong, he has virtual access to a team of experts that can help him fix the system. While AI may sometimes be able to provide suggestions for how to fix itself, it seems unlikely that it could do a good job of this for every possible machine problem. Humans are necessary to fix AI systems on occasion, and human fixers will have long-term employment.
Leading organizational change management. Several of our cases illustrate the need for organizational change if AI systems are going to be used effectively, and using an AI-based organizational change management system itself to perform those types of tasks seems unlikely even under the most optimistic scenarios.
At Southern California Edison, an AI system identified field service tasks done by employees servicing the electrical grid that were safety risks. Long-term education and lobbying were required for the system to be adopted by stakeholders. Similarly, at Morgan Stanley, the company made financial advisers’ use of the “next best action” AI system completely voluntary, and the company stimulated adoption by exercising informal persuasion, removing obstacles to usage, and internally publicizing measures showing that those advisers who used the system had better financial performance. Only humans can convince stakeholders to adopt a new approach, coordinate complex cross-functional change, and understand why humans are resisting the use of a new system.
Providing job satisfaction and nurturing morale. At Radius Financial Group, a home mortgage company, we observed that employees had high morale and employee job satisfaction despite heavy use of AI and automation and close monitoring of individual performance with data and analytics. People told us that they liked their jobs because of the other people who worked there.
This echoed other comments we heard elsewhere and suggests that it’s the human element of management and collegial relationships that lead to positive emotions about work. A company’s approach to human engagement can counter any “dehumanizing” impacts of AI and other technologies.
What to Do About AI Limitations and Human Strengths
The implications of having areas in the work organization in which humans are better than AI — at least thus far — are perhaps obvious. If AI is to make decisions, in most cases it should be possible for humans to override the decisions, as Stitch Fix can with its AI-based clothing recommendations. Organizations may want to monitor the human overrides and assess their frequency and effectiveness. This type of evaluation can help both the system designers and the employees partnering with the AI support tool.
AI experts should spend time with managers and employees and explain what AI can and can’t do. These should be application- and domain-specific discussions since AI’s capabilities are so broad and can be used in so many different ways. The experts and the managers together should, in most cases, ease AI systems into a job task-by-task as opposed to taking any sort of “big bang” approach. It’s also not a bad idea to, as Morgan Stanley did, give employees some say in whether and when they adopt AI capabilities in their jobs, at least in the initial phases of deployment.
All those involved in AI, including developers and potential users and their managers, should understand that AI implementation is a change management activity. They should budget for change management assessments and interventions, and ensure that the necessary change management skills are available on teams. If human jobs are planned to be eliminated by AI, it is probably wise to move slowly in implementing that type of change. Ethical management, of course, also requires that employers are transparent with employees about future job prospects and how AI might affect them so that employees can prepare themselves for value-adding roles to AI or seek employment alternatives. However, we have found large-scale job loss from AI to be quite rare, and we believe most organizations benefit more from human-machine collaboration than from substituting machines for humans.