Why AI's Future Depends on Human Ingenuity
As we move into 2025, companies will need to create AI solutions that foster positive, productive interactions, ensuring technology works alongside people ethically and effectively.
News
- AI Strategy Now Critical to CEO Survival, New Report Reveals
- DeepSeek Fails 58% of the Jailbreak Tests by Qualys TotalAI
- Businesses Face Significant AI Adoption Gap, Survey Finds
- Gartner Identifies Key Data and Analytics Trends Shaping 2025
- GovTech Conclave 2025: Shaping the Future of Digital Governance in the Middle East
- UAE to employ AI to combat and penalize consumer fraud
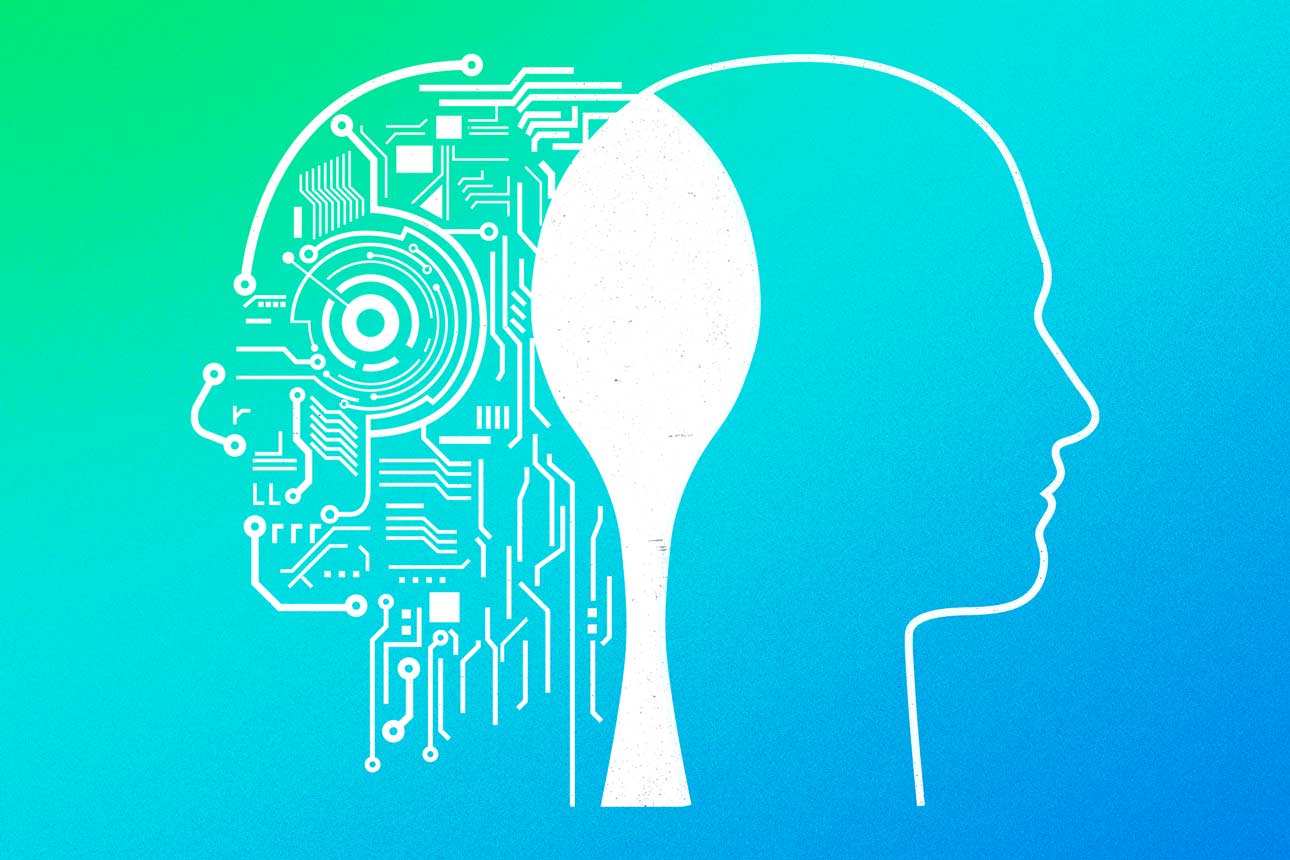
[Image source: Krishna Prasad/MITSMR Middle East]
Artificial intelligence (AI) is not a new concept; its roots trace back decades. John McCarthy and Marvin Minsky introduced the term during the Dartmouth Summer Research Project on Artificial Intelligence in 1956. While progress was initially gradual, the 2000s ushered in a turning point with substantial investments that accelerated AI development.
In 2023, generative AI (GenAI) tools like OpenAI’s ChatGPT-4 brought AI into the mainstream and became central to public discourse and business strategy. Organizations worldwide began to explore AI’s potential for streamlining processes, reducing errors, cutting costs through automation, and uncovering insights within vast data sets.
However, by 2024, the conversation shifted from excitement to action. Governments, businesses, and the public adopted a more measured approach, carefully considering AI’s broader implications. While some paused to reassess their strategies, others embraced sandbox experimentation or integrated AI into specific areas of their operations.
The focus also turned toward regulations—both international and domestic. Governments worldwide began prioritizing consumer protection, civil liberties, intellectual property rights, and fair business practices as they worked to regulate AI.
- Across the EU, the landmark AI Act—now in force—emphasizes transparency and accountability, while updated data protection guidelines stress limits on data collection.
- In the UK, an AI Opportunities Action Plan was published, outlining a roadmap towards an AI Bill that aims to harness AI’s potential for economic growth, enhance public services, and improve AI adoption foundations.
- In the US, a proposed Deepfake Bill targets synthetic content, mandating watermarks and stronger content integrity tools. Meanwhile, the Treasury is exploring AI’s growing influence on financial services.
- In China, ambitious guidelines aim to establish 50 national AI standards by 2026, prioritizing sustainability and talent development.
- In the UAE, AI Charter ensures safe and fair AI development.
This global movement reflected a growing consensus: AI’s potential must be balanced with safeguards to protect society, promote fairness, and foster innovation responsibly. Its success depends on innovation and how it integrates with human expertise and decision-making. It is equally important to ensure AI enhances rather than replaces human capabilities for a future where technology serves humanity.
In 2025, the role of humans as the catalysts in the adoption and strengthening of AI will only become stronger, and companies will have to ensure that their AI solutions promote positive and productive interactions between humans and machines.
Human-machine collaboration
“To make ethical AI solutions that promote positive and productive interactions between humans and machines, you need to first enable ethical humans,” Mark Gibbs, EMEA President, UiPath.
Integrating powerful automation and AI capabilities, focusing on security, governance, and risk, ensures these technologies are deployed responsibly. When decisions require human judgment, involving people in the automation process enables informed choices and seamless collaboration between humans and machines.
A key principle in this approach is democratizing emerging technologies and providing users with the tools to understand and use them effectively.
According to Alexey Sidorov, Data Science guru and evangelist at Denodo, “To ensure AI solutions are ethical and transparent, it’s important to use data virtualization technology and design algorithms that are free from bias and aligned with societal values.”
Himanshu Gupta, CTO and Co-Founder of Shipsy, a logistics software solution provider, says focusing on “user-centered design” is important. This makes AI systems intuitive, enhances human decision-making rather than replacing it, and prioritizes clear communication between systems and users. Features like real-time dashboards and actionable alerts play a key role in effectively presenting insights.
Both Gibbs and Gupta stress the need for staff training and upskilling to help teams adapt to AI integration. “Continuous improvement requires a feedback loop,” Gupta notes, adding that ongoing evaluation helps AI systems perform seamlessly in real-world operations.
Humans should remain “in the driver’s seat” with respect to the decision-making process, viewing AI systems as human-enabling tools rather than replacements, adds Gibbs. He outlines a few decision points for human intervention, which include:
- Uncertainty Detection: AI systems employ guardrails and tests to account for uncertainties in AI models. When issues are flagged, the system requests human review to ensure accuracy and reliability.
- Flexibility in Model Integration: AI solutions allow customers to integrate multiple models, user interfaces, and APIs, allowing them to design workflows with appropriate human intervention points based on their specific needs and risk tolerance.
- Workflow Analyzer and Automation Ops: Tools embedded in AI systems help customers govern their automation, providing the ability to define and monitor human intervention points to ensure smooth operations.
Ongoing monitoring of human-in-the-loop processes helps assess the impact of such interventions. Key indicators include:
- Accuracy Improvements: Evaluating how human input enhances the accuracy of AI outcomes.
- Reduction in Errors: Analyzing how human intervention helps reduce biases or errors in the system.
- Process Completion Times and Efficiency: Monitoring how interventions affect the speed and efficiency of processes.
- User Satisfaction and Trust: Measuring user trust and satisfaction ensures the AI system is effective and ethical.
Safety, Reliability, and Bias in AI systems
Gibbs advocates an open AI approach: “Users should be able to combine the best of various AI models, whether general or specialized.” Transparency is a cornerstone of this approach, ensuring users can make informed and ethical deployment decisions. Trust-building through clear visibility into AI processes helps users confidently navigate options.
Sidorov stresses “safety and reliability through rigorous data governance and security protocols”. Continuous monitoring maintains data integrity and ensures role-based access to sensitive information, protecting systems while upholding accountability. Addressing algorithmic bias requires regular audits, adherence to ethical practices, and continuous output monitoring to ensure fairness and alignment with industry standards.
Talking about the importance of testing and validation in building trustworthy AI, Gupta says simulations and real-world trials identify and resolve issues before deployment, while ongoing performance monitoring helps systems adapt to new data. To address bias, he highlights using diverse datasets and fairness-aware algorithms to identify and correct unintended biases. “Explainable AI processes,” Gupta adds, “allow stakeholders to understand decisions and outcomes, building trust.”
Gibbs suggests the following processes and tools to identify and correct bias in AI models:
- Data quality assessment:High-quality, lawfully sourced, and securely managed data are foundational to building effective tools. By addressing potential issues at the data collection stage, this approach minimizes the risk of bias entering the system.
- Model rating feature: A built-in model rating capability simplifies the process of model validation. By assigning scores for performance, accuracy, and balance, it enables users to identify potential bias issues with minimal manual effort.
- Guided improvement experience: Following the model rating process, a step-by-step improvement framework supports users in refining their models. This includes actionable guidance on addressing any detected bias, fostering more reliable outcomes.
- Active review process: An active review process continuously evaluates for bias and other critical factors, reinforcing the commitment to developing trustworthy AI systems.
- Real-World Applications: Automated model rating features in some AI platforms help users—especially those without extensive AI expertise—understand when a model is adequately trained and balanced. By combining automated validation with clear improvement steps, these tools enable proactive bias mitigation, ensuring AI systems perform as intended without unintended consequences.
Data Privacy and Transparency
Data privacy and ethical AI implementation are critical considerations for modern platforms.
“Data privacy is maintained through encryption—both in transit and at rest—safeguarding sensitive information from unauthorized access. We practice data minimization, collecting only the necessary data to reduce exposure risks, while anonymization and pseudonymization further protect individual privacy.” Compliance with regulations, such as the UAE’s National Strategy for Artificial Intelligence 2031, is a key priority, ensuring all data handling adheres to legal standards.
Regular legal audits, expert consultations, and stringent data protection measures help companies align with global standards. Gupta highlights the need for ongoing training to ensure teams know legal requirements and best practices.
Gibbs highlights how organizations can address these concerns effectively through governance, transparency, and secure integration practices.
- Third-party Data Agreements: Agreements with all third-party data sub-processors, including providers of large language models (LLMs) should prohibit the use of customer data for model training. This ensures sensitive information remains protected and used solely for its intended purpose.
- Data Integrity and Security: Treat all data interacting with the products and third-party LLMs with the utmost integrity, security, and privacy.
- Audit Trails: Comprehensive audit trails ensure ethical and compliant use of Gen AI features, tracking who accessed data, when, and how. Customers have complete visibility into using Gen AI features, models, and data transfers between the platform and third-party models.
Platforms can also employ both specialized AI and Gen AI in their offerings:
- Specialized AI: These implementations are less error-prone, as model training and rigorous testing are performed before release. Specialized AI models interpret and label data but do not generate new data from prompts. Customers can refine these models further through additional training.
- Generative AI: Private Gen AI models are provided via the AI Trust Layer, enabling customers to securely use services like Azure OpenAI. These private models avoid training on customer data and are less error-prone than public models.
All the AI features include a human-in-the-loop option, allowing users to review and edit predictions before automation, ensuring accuracy and reducing errors.
Sidorov suggests maintaining data privacy and transparency through:
- Data Governance Frameworks: Robust frameworks and stringent access controls safeguard sensitive information.
- Secure, Role-based Access: Role-based access systems further enhance security by ensuring that only authorized individuals can interact with sensitive datasets.
- Transparency: Comprehensive data lineage and audit trails enable stakeholders to trace data sources and transformations seamlessly.
Protecting Customer Data with Third-Party AI Models
Several protocols, as outlined by Gibbs, can be implemented to ensure that customer data is fully protected when interfacing with third-party AI models:
- Open Ecosystem with Clear Labeling: An open approach to AI allows users to select from user-contributed, or models from external providers. AI capabilities are clearly labeled to ensure customers can make informed decisions about using the technology, including third-party models.
- Strong Evaluation Standards: Strong standards have been developed to evaluate third-party AI and large language model (LLM) technologies, focusing on assessing their data protection practices.
- Data Protection Measures: Appropriate measures are taken to protect data processed within the platform. These measures extend to data shared with third-party models.
- Legal and Compliant Data Collection: Emphasis is placed on the importance of lawful data sourcing and secure management, particularly with regard to data shared with or processed by third-party models.
Compliance can be ensured through:
- Regular Security Assessments: Regular security evaluations of third-party integrations to maintain data protection standards.
- Contractual Obligations: Clear data protection obligations are outlined in contracts with third-party providers.
- Ongoing Monitoring: Continuous monitoring of data flows to and from third-party models ensures adherence to data protection policies.
Fostering Continuous Learning and Inclusivity in AI
To ensure that humans remain in the driver’s seat when implementing AI, it is crucial to prioritize continuous education and learning. As AI increasingly integrates into various industries, human expertise remains essential for making ethical decisions, interpreting complex data, and ensuring AI systems align with societal values. In human-in-the-loop systems, where AI assists human decision-making, continuous learning allows individuals to better understand, leverage, and control these technologies effectively. This ongoing education ensures that human control over AI-driven processes is maintained and systems evolve responsibly.
A key strategy for fostering continuous learning is encouraging engagement with a variety of educational resources, such as industry conferences, webinars, and online courses. By participating in these forums, professionals can learn from thought leaders and peers, gaining insights into the latest advancements. Internally, organizations should cultivate a culture of knowledge sharing, supporting initiatives like tech talks, hackathons, and collaborative projects. These spaces allow teams to experiment with AI technologies and apply them to real-world challenges. Creating innovation labs or providing hands-on environments further empowers employees to explore AI-driven solutions, turning theoretical knowledge into practical expertise.
“We conduct regular AI workshops open to all employees, regardless of their technical background, to demystify AI concepts and encourage broader understanding,” says Gupta.
In addition to fostering internal learning, organizations should recognize the importance of providing accessible education for a broader community. Offering free online courses and industry-recognized certifications allows individuals to gain skills in AI and automation, democratizing access to expertise.
Equally important is promoting diversity within AI development. A diverse team is better equipped to identify blind spots, ensure broader perspectives in decision-making, and enhance creativity.
“We need to broaden the definition of diversity. We tend to think of diversity mostly in the context of race, gender, ethnicity, sexual identity, and religion. But it is also critical to give opportunities to people whose resumes may not fully align with job descriptions. In other words, seek out diversity in experience, too. Fresh perspectives from people with varied, cross-functional experience can spell the difference between stagnation and innovation,” adds Gibbs.
Despite technological advancements, human intervention remains critical in AI operations. From reviewing AI predictions to refining algorithms, human oversight ensures accuracy, fairness, and accountability. As Gupta emphasizes, fostering collaboration across cross-functional teams is essential to challenge assumptions and create equitable AI solutions.